Quasideterminant: Difference between revisions
en>R.e.b. |
en>Yobot m clean up, References after punctuation per WP:REFPUNC and WP:CITEFOOT using AWB (8797) |
||
Line 1: | Line 1: | ||
In [[statistics]] and [[signal processing]], the '''orthogonality principle''' is a necessary and sufficient condition for the optimality of a [[Bayesian estimator]]. Loosely stated, the orthogonality principle says that the error vector of the optimal estimator (in a [[mean square error]] sense) is orthogonal to any possible estimator. The orthogonality principle is most commonly stated for linear estimators, but more general formulations are possible. Since the principle is a necessary and sufficient condition for optimality, it can be used to find the [[minimum mean square error]] estimator. | |||
== Orthogonality principle for linear estimators == | |||
The orthogonality principle is most commonly used in the setting of linear estimation.<ref>Kay, p.386</ref> In this context, let ''x'' be an unknown [[random vector]] which is to be estimated based on the observation vector ''y''. One wishes to construct a linear estimator <math>\hat{x} = Hy + c</math> for some matrix ''H'' and vector ''c''. Then, the orthogonality principle states that an estimator <math>\hat{x}</math> achieves [[minimum mean square error]] if and only if | |||
* <math>E \{ (\hat{x} - x ) y^T \} = 0,</math> and | |||
* <math>E \{ \hat{x} - x \} = 0.</math> | |||
If ''x'' and ''y'' have zero mean, then it suffices to require the first condition. | |||
=== Example === | |||
Suppose ''x'' is a [[Gaussian random variable]] with mean ''m'' and variance <math>\sigma_x^2.</math> Also suppose we observe a value <math>y=x+w,</math> where ''w'' is Gaussian noise which is independent of ''x'' and has mean 0 and variance <math>\sigma_w^2.</math> We wish to find a linear estimator <math>\hat{x} = hy+c</math> minimizing the MSE. Substituting the expression <math>\hat{x} = hy + c</math> into the two requirements of the orthogonality principle, we obtain | |||
: <math>0 = E \{ (\hat{x} - x ) y \}</math> | |||
: <math>0 = E \{ (hx+hw+c-x)(x+w) \}</math> | |||
: <math>0 = h (\sigma_x^2+\sigma_w^2) + cm - \sigma_x^2</math> | |||
and | |||
: <math>0 = E \{ \hat{x} - x \}</math> | |||
: <math>0 = E \{ hx+hw+c-x \}</math> | |||
: <math>0 = (h-1)m + c .</math> | |||
Solving these two linear equations for ''h'' and ''c'' results in | |||
: <math> h = \frac{\sigma_x^2 - m^2}{(\sigma_x^2 - m^2)+\sigma_w^2}, \quad c = \frac{\sigma_w^2}{(\sigma_x^2 - m^2)+\sigma_w^2} m , </math> | |||
so that the linear minimum mean square error estimator is given by | |||
: <math> \hat{x} = \frac{\sigma_x^2 - m^2}{(\sigma_x^2 - m^2)+\sigma_w^2} y + \frac{\sigma_w^2}{(\sigma_x^2 - m^2)+\sigma_w^2} m.</math> | |||
This estimator can be interpreted as a weighted average between the noisy measurements ''y'' and the prior expected value ''m''. If the noise variance <math>\sigma_w^2</math> is low compared with the variance of the prior minus the squared mean <math>\sigma_x^2 - m^2</math> (corresponding to a high [[signal to noise ratio|SNR]]), then most of the weight is given to the measurements ''y'', which are deemed more reliable than the prior information. Conversely, if the noise variance is relatively higher, then the estimate will be close to ''m'', as the measurements are not reliable enough to outweigh the prior information. | |||
Finally, note that because the variables ''x'' and ''y'' are jointly Gaussian, the minimum MSE estimator is linear.<ref>See the article [[minimum mean square error]].</ref> Therefore, in this case, the estimator above minimizes the MSE among all estimators, not only linear estimators. | |||
== General formulation == | |||
Let <math>V</math> be a [[Hilbert space]] of random variables with an [[inner product]] defined by <math>\langle x,y \rangle = E \{ x^H y \}</math>. Suppose <math>W</math> is a [[closed set|closed]] subspace of <math>V</math>, representing the space of all possible estimators. One wishes to find a vector <math>\hat{x} \in W</math> which will approximate a vector <math>x \in V</math>. More accurately, one would like to minimize the mean squared error (MSE) <math>E \| x - \hat{x} \|^2</math> between <math>\hat{x}</math> and <math>x</math>. | |||
In the special case of linear estimators described above, the space <math>V</math> is the set of all functions of <math>x</math> and <math>y</math>, while <math>W</math> is the set of linear estimators, i.e., linear functions of <math>y</math> only. Other settings which can be formulated in this way include the subspace of [[causal filter|causal]] linear filters and the subspace of all (possibly nonlinear) estimators. | |||
Geometrically, we can see this problem by the following simple case where <math>W</math> is a [[dimension (vector space)|one-dimensional]] subspace: | |||
[[Image:Orthogonality principle.png|350px|center]] | |||
We want to find the closest approximation to the vector <math>x</math> by a vector <math>\hat{x}</math> in the space <math>W</math>. From the geometric interpretation, it is intuitive that the best approximation, or smallest error, occurs when the error vector, <math>e</math>, is orthogonal to vectors in the space <math>W</math>. | |||
More accurately, the general orthogonality principle states the following: Given a closed subspace <math>W</math> of estimators within a Hilbert space <math>V</math> and an element <math>x</math> in <math>V</math>, an element <math>\hat{x} \in W</math> achieves minimum MSE among all elements in <math>W</math> if and only if <math>E \{ (x-\hat{x}) y^T \} = 0 </math> for all <math>y \in W.</math> | |||
Stated in such a manner, this principle is simply a statement of the [[Hilbert projection theorem]]. Nevertheless, the extensive use of this result in signal processing has resulted in the name "orthogonality principle." | |||
== A solution to error minimization problems == | |||
The following is one way to find the [[minimum mean square error]] estimator by using the orthogonality principle. | |||
We want to be able to approximate a vector <math>x</math> by | |||
: <math>x=\hat{x}+e\,</math> | |||
where | |||
: <math>\hat{x}=\sum_i c_{i}p_{i}</math> | |||
is the approximation of <math>x</math> as a linear combination of vectors in the subspace <math>W</math> spanned by <math>p_{1},p_{2},\ldots.</math> Therefore, we want to be able to solve for the coefficients, <math>c_{i}</math>, so that we may write our approximation in known terms. | |||
By the orthogonality theorem, the square norm of the error vector, <math>\left\Vert e\right\Vert ^{2}</math>, is minimized when, for all ''j'', | |||
: <math>\left\langle x-\sum_i c_{i}p_{i},p_{j}\right\rangle =0.</math> | |||
Developing this equation, we obtain | |||
: <math> | |||
\left\langle x,p_{j}\right\rangle =\left\langle \sum_i c_{i}p_{i},p_{j}\right\rangle =\sum_i c_{i}\left\langle p_{i},p_{j}\right\rangle.</math> | |||
If there is a finite number <math>n</math> of vectors <math>p_i</math>, one can write this equation in matrix form as | |||
: <math> | |||
\begin{bmatrix} | |||
\left\langle x,p_{1}\right\rangle \\ | |||
\left\langle x,p_{2}\right\rangle \\ | |||
\vdots\\ | |||
\left\langle x,p_{n}\right\rangle \end{bmatrix} | |||
= | |||
\begin{bmatrix} | |||
\left\langle p_{1},p_{1}\right\rangle & \left\langle p_{2},p_{1}\right\rangle & \cdots & \left\langle p_{n},p_{1}\right\rangle \\ | |||
\left\langle p_{1},p_{2}\right\rangle & \left\langle p_{2},p_{2}\right\rangle & \cdots & \left\langle p_{n},p_{2}\right\rangle \\ | |||
\vdots & \vdots & \ddots & \vdots\\ | |||
\left\langle p_{1},p_{n}\right\rangle & \left\langle p_{2},p_{n}\right\rangle & \cdots & \left\langle p_{n},p_{n}\right\rangle \end{bmatrix} | |||
\begin{bmatrix} | |||
c_{1}\\ | |||
c_{2}\\ | |||
\vdots\\ | |||
c_{n}\end{bmatrix}.</math> | |||
Assuming the <math>p_i</math> are [[linearly independent]], the [[Gramian matrix]] can be inverted to obtain | |||
: <math>\begin{bmatrix} | |||
c_{1}\\ | |||
c_{2}\\ | |||
\vdots\\ | |||
c_{n}\end{bmatrix} | |||
= | |||
\begin{bmatrix} | |||
\left\langle p_{1},p_{1}\right\rangle & \left\langle p_{2},p_{1}\right\rangle & \cdots & \left\langle p_{n},p_{1}\right\rangle \\ | |||
\left\langle p_{1},p_{2}\right\rangle & \left\langle p_{2},p_{2}\right\rangle & \cdots & \left\langle p_{n},p_{2}\right\rangle \\ | |||
\vdots & \vdots & \ddots & \vdots\\ | |||
\left\langle p_{1},p_{n}\right\rangle & \left\langle p_{2},p_{n}\right\rangle & \cdots & \left\langle p_{n},p_{n}\right\rangle \end{bmatrix}^{-1} | |||
\begin{bmatrix} | |||
\left\langle x,p_{1}\right\rangle \\ | |||
\left\langle x,p_{2}\right\rangle \\ | |||
\vdots\\ | |||
\left\langle x,p_{n}\right\rangle \end{bmatrix},</math> | |||
thus providing an expression for the coefficients <math>c_i</math> of the minimum mean square error estimator. | |||
==See also== | |||
*[[Minimum mean square error]] | |||
*[[Hilbert projection theorem]] | |||
== Notes == | |||
{{reflist}} | |||
== References == | |||
* {{cite book | |||
| last = Kay | |||
| first = S. M. | |||
| title = Fundamentals of Statistical Signal Processing: Estimation Theory | |||
| year = 1993 | |||
| publisher = Prentice Hall | |||
| isbn = 0-13-042268-1 }} | |||
* {{Cite document | last=Moon | first=Todd K. | title = Mathematical Methods and Algorithms for Signal Processing | year = 2000 | publisher = Prentice-Hall | postscript=<!--None--> | isbn=0-201-36186-8}} | |||
[[Category:Estimation theory]] | |||
[[Category:Statistical principles]] |
Revision as of 19:45, 11 December 2012
In statistics and signal processing, the orthogonality principle is a necessary and sufficient condition for the optimality of a Bayesian estimator. Loosely stated, the orthogonality principle says that the error vector of the optimal estimator (in a mean square error sense) is orthogonal to any possible estimator. The orthogonality principle is most commonly stated for linear estimators, but more general formulations are possible. Since the principle is a necessary and sufficient condition for optimality, it can be used to find the minimum mean square error estimator.
Orthogonality principle for linear estimators
The orthogonality principle is most commonly used in the setting of linear estimation.[1] In this context, let x be an unknown random vector which is to be estimated based on the observation vector y. One wishes to construct a linear estimator for some matrix H and vector c. Then, the orthogonality principle states that an estimator achieves minimum mean square error if and only if
If x and y have zero mean, then it suffices to require the first condition.
Example
Suppose x is a Gaussian random variable with mean m and variance Also suppose we observe a value where w is Gaussian noise which is independent of x and has mean 0 and variance We wish to find a linear estimator minimizing the MSE. Substituting the expression into the two requirements of the orthogonality principle, we obtain
and
Solving these two linear equations for h and c results in
so that the linear minimum mean square error estimator is given by
This estimator can be interpreted as a weighted average between the noisy measurements y and the prior expected value m. If the noise variance is low compared with the variance of the prior minus the squared mean (corresponding to a high SNR), then most of the weight is given to the measurements y, which are deemed more reliable than the prior information. Conversely, if the noise variance is relatively higher, then the estimate will be close to m, as the measurements are not reliable enough to outweigh the prior information.
Finally, note that because the variables x and y are jointly Gaussian, the minimum MSE estimator is linear.[2] Therefore, in this case, the estimator above minimizes the MSE among all estimators, not only linear estimators.
General formulation
Let be a Hilbert space of random variables with an inner product defined by . Suppose is a closed subspace of , representing the space of all possible estimators. One wishes to find a vector which will approximate a vector . More accurately, one would like to minimize the mean squared error (MSE) between and .
In the special case of linear estimators described above, the space is the set of all functions of and , while is the set of linear estimators, i.e., linear functions of only. Other settings which can be formulated in this way include the subspace of causal linear filters and the subspace of all (possibly nonlinear) estimators.
Geometrically, we can see this problem by the following simple case where is a one-dimensional subspace:
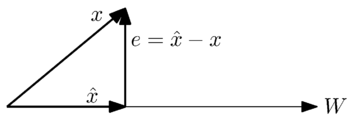
We want to find the closest approximation to the vector by a vector in the space . From the geometric interpretation, it is intuitive that the best approximation, or smallest error, occurs when the error vector, , is orthogonal to vectors in the space .
More accurately, the general orthogonality principle states the following: Given a closed subspace of estimators within a Hilbert space and an element in , an element achieves minimum MSE among all elements in if and only if for all
Stated in such a manner, this principle is simply a statement of the Hilbert projection theorem. Nevertheless, the extensive use of this result in signal processing has resulted in the name "orthogonality principle."
A solution to error minimization problems
The following is one way to find the minimum mean square error estimator by using the orthogonality principle.
We want to be able to approximate a vector by
where
is the approximation of as a linear combination of vectors in the subspace spanned by Therefore, we want to be able to solve for the coefficients, , so that we may write our approximation in known terms.
By the orthogonality theorem, the square norm of the error vector, , is minimized when, for all j,
Developing this equation, we obtain
If there is a finite number of vectors , one can write this equation in matrix form as
Assuming the are linearly independent, the Gramian matrix can be inverted to obtain
thus providing an expression for the coefficients of the minimum mean square error estimator.
See also
Notes
43 year old Petroleum Engineer Harry from Deep River, usually spends time with hobbies and interests like renting movies, property developers in singapore new condominium and vehicle racing. Constantly enjoys going to destinations like Camino Real de Tierra Adentro.
References
- 20 year-old Real Estate Agent Rusty from Saint-Paul, has hobbies and interests which includes monopoly, property developers in singapore and poker. Will soon undertake a contiki trip that may include going to the Lower Valley of the Omo.
My blog: http://www.primaboinca.com/view_profile.php?userid=5889534 - Template:Cite document
- ↑ Kay, p.386
- ↑ See the article minimum mean square error.