Ultrasensitivity: Difference between revisions
en>Rjwilmsi m →Switching Behavior of GTPases: Journal cites, completed 1 page range, using AWB (8087) |
grammar |
||
Line 1: | Line 1: | ||
[[Image:YYL1.png|right|thumb|Controlling a simple network.]] | |||
'''Network Controllability''' is concerned about the structural [[controllability]] of a [[Graph (mathematics)|network]]. Controllability describes our ability to guide a dynamical system from any initial state to any desired final state in finite time, with a suitable choice of inputs. This definition agrees well with our intuitive notion of control. The controllability of general directed and weighted complex networks has recently been the subject of intense study by a number of groups, worldwide. | |||
==Background== | |||
Consider the canonical linear time-invariant dynamics on a complex network | |||
<math> | |||
\dot{\mathbf{X}}(t) = \mathbf{A} \cdot \mathbf{X}(t) + \mathbf{B}\cdot \mathbf{u}(t) | |||
</math> | |||
where the vector <math>\mathbf{X}(t)=(x_1(t),\cdots,x_N(t))^\mathrm{T}</math> captures the state of a system of <math>N</math> nodes at time <math>t</math>. The <math>N \times N</math> | |||
matrix <math>\mathbf{A}</math> describes the system's wiring digram and the interaction strength between the components. The <math>N \times M</math> matrix <math>\mathbf{B}</math> identifies the nodes controlled by an outside controller. The system is controlled through the time dependent input vector <math>\mathbf{u}(t) = (u_1(t),\cdots,u_M(t))^\mathrm{T}</math> that the controller imposes on the system. To identify the ''minimum'' number of driver nodes, denoted by <math>N_\mathrm{D}</math>, whose control is sufficient to fully control the system's dynamics, Liu et al.<ref name="Liu-Nature-11">Y.-Y. Liu, J.-J. Slotine, A.-L. Barabási, ''Nature'' '''473''' (2011).</ref> attempted to combine the tools from structural control theory, graph theory and statistical physics. They showed<ref name="Liu-Nature-11"/> that the minimum number of inputs or driver nodes needed to maintain full control of the network is determined by the 'maximum matching’ in the network, that is, the maximum set of links that do not share start or end nodes. They tried<ref name="Liu-Nature-11"/> to develop an analytical framework, based on the in-out degree distribution, which predicts <math>n_\mathrm{D} =N_\mathrm{D}/N </math> for scale-free and Erdős–Rényi Graphs. It is however notable, that their formulation<ref name="Liu-Nature-11"/> would predict same values of <math>{n_\mathrm{D}}</math> for a chain graph and for a weak densely connected graph. Obviously, both these graphs have very different in and out degree distributions. A recent unpublished work,<ref name = "Arxiv_Close_Betw">SJ Banerjee and S Roy, ARXIV:1209.3737</ref> questions whether [[Degree (graph theory)|degree]], which is a purely local measure in networks, would completely describe controllability and whether even slightly distant nodes would have no role in deciding network controllability. Indeed, for many real-word networks, namely, food webs, neuronal and metabolic networks, the mismatch in values of <math>{n_\mathrm{D}}^{real}</math> and <math>{n_\mathrm{D}}^\mathrm{rand\_degree}</math> calculated by Liu et al.<ref name="Liu-Nature-11"/> is notable. It is obvious that if controllability is decided mainly by degree, why are <math>{n_\mathrm{D}}^{real}</math> and <math>{n_\mathrm{D}}^\mathrm{rand\_degree}</math> so different for many real world networks? They argued <ref name="Liu-Nature-11"/> (arXiv:1203.5161v1), that this might be due to the effect of degree correlations. However, it has been shown<ref name = "Arxiv_Close_Betw"/> that network controllability can be altered only by using [[betweenness centrality]] and [[closeness centrality]], without using [[degree (graph theory)]] or degree correlations at all. | |||
[[File:YYL2.pdf|right|thumb|A schematic digram shows the control of a directed network. For a given directed network (Fig. a), one calculates its maximum matching: a largest set of edges without common heads or tails. The maximum matching will compose of a set of vertex-disjoint directed paths and directed cycles (see red edges in Fig.b). If a node is a head of a matching edge, then this node is matched (green nodes in Fig.b). Otherwise, it is unmatched (white nodes in Fig.b). Those unmatched nodes are the nodes one needs to control, i.e. the driver nodes. By injecting signals to those driver nodes, one gets a set of directed path with starting points being the inputs (see Fig.c). Those paths are called "stems". The resulting digraph is called U-rooted factorial connection. By "grafting" the directed cycles to those "stems", one gets "buds". The resulting digraph is called the cacti (see Fig.d). According to the structural controllability theorem,<ref name="Lin-74"/> since there is a cacti structure spanning the controlled network (see Fig.e), the system is controllable. The cacti structure (Fig.d) underlying the controlled network (Fig.e) is the "skeleton" for maintaining controllability.]] | |||
===Structural Controllability=== | |||
The concept of the structural properties was first introduced by Lin (1974)<ref name="Lin-74">C.-T. Lin, ''IEEE Trans. Auto. Contr.'' '''19''' | |||
(1974).</ref> and then extended by Shields and Pearson (1976)<ref name="Shields-76">R. W. Shields and J. B. Pearson, ''IEEE Trans. Auto. Contr.'' '''21''' | |||
(1976).</ref> and alternatively derived by Glover and Silverman (1976).<ref name="Glover-76">K. Glover and L. M. Silverman, ''IEEE Trans. Auto. Contr.'' '''21''' | |||
(1976).</ref> The main question is whether the lack of controllability or observability are generic with respect to the variable system parameters. In the framework of structural control the system parameters are either independent free variables or fixed zeros. This is consistent for models of physical systems since parameter values are never known exactly, with the exception of zero values which express the absence of interactions or connections. | |||
===Maximum Matching=== | |||
In graph theory, a [[matching (graph theory)|matching]] is a set of edges without common vertices. Liu et al.<ref name="Liu-Nature-11"/> extended this definition to directed graph, where a matching is a set of directed edges that do not share start or end vertices. It is easy to check that a matching of a directed graph composes of a set of vertex-disjoint simple paths and cycles. The maximum matching of a directed network can be efficiently calculated by working in the bipartite representation using the classical [[Hopcroft–Karp algorithm]], which runs in O(''E''√N) time in the worst case. For undirected graph, analytical solutions of the size and number of maximum matchings have been studied using the [[cavity method]] developed in statistical physics.<ref name="Zdeborova-06">L. Zdeborova and M. Mezard, ''J. Stat. Mech.'' '''05''' (2006).</ref> Liu et al.<ref name="Liu-Nature-11"/> extended the calculations for directed graph. | |||
By calculating the maximum matchings of a wide range of real networks, Liu et al.<ref name="Liu-Nature-11"/> asserted that the number of driver nodes is determined mainly by the networks degree distribution <math>P(k_\mathrm{in}, k_\mathrm{out})</math>. They also calculated the average number of driver nodes for a network ensemble with arbitrary degree distribution using the [[cavity method]]. It is interesting that for a chain graph and a weak densely connected graph, both of which have very different in and out degree distributions; the formulation of Liu et al.<ref name="Liu-Nature-11"/> would predict same values of <math>{n_\mathrm{D}}</math>. Also, for many real-word networks, namely, food webs, neuronal and metabolic networks, the mismatch in values of <math>{n_\mathrm{D}}^{real}</math> and <math>{n_\mathrm{D}}^\mathrm{rand\_degree}</math> calculated by Liu et al.<ref name="Liu-Nature-11"/> is notable. If controllability is decided purely by degree, why are <math>{n_\mathrm{D}}^{real}</math> and <math>{n_\mathrm{D}}^\mathrm{rand\_degree}</math> so different for many real world networks? It remains open to scrutiny whether ''control robustness" in networks is influenced more by using [[betweenness centrality]] and [[closeness centrality]]<ref name = "Arxiv_Close_Betw"/> over [[degree (graph theory)]] based metrics. | |||
While sparser graphs are more difficult to control,<ref name="Liu-Nature-11"/><ref name = "Arxiv_Close_Betw"/> it would obviously be interesting to find whether [[betweenness centrality]] and [[closeness centrality]]<ref name = "Arxiv_Close_Betw"/> or degree heterogeneity<ref name="Liu-Nature-11"/> plays a more important role in deciding controllability of sparse graphs with almost similar degree distributions. | |||
==Future Directions== | |||
This recent immense activity inspires hope of new breakthroughs in structural controllability of complex networks. | |||
== See also == | |||
* [[Controllability Gramian]] | |||
==References== | |||
<references/> | |||
== External links == | |||
* [http://barabasilab.neu.edu/projects/controllability/ The network controllability project website] | |||
* [http://www.youtube.com/watch?v=9-q2qpOJfkg The video showing network controllability] | |||
<!--- Categories ---> | |||
[[Category:Articles created via the Article Wizard]] | |||
[[Category:Network theory]] |
Revision as of 01:49, 15 December 2013

Network Controllability is concerned about the structural controllability of a network. Controllability describes our ability to guide a dynamical system from any initial state to any desired final state in finite time, with a suitable choice of inputs. This definition agrees well with our intuitive notion of control. The controllability of general directed and weighted complex networks has recently been the subject of intense study by a number of groups, worldwide.
Background
Consider the canonical linear time-invariant dynamics on a complex network where the vector captures the state of a system of nodes at time . The matrix describes the system's wiring digram and the interaction strength between the components. The matrix identifies the nodes controlled by an outside controller. The system is controlled through the time dependent input vector that the controller imposes on the system. To identify the minimum number of driver nodes, denoted by , whose control is sufficient to fully control the system's dynamics, Liu et al.[1] attempted to combine the tools from structural control theory, graph theory and statistical physics. They showed[1] that the minimum number of inputs or driver nodes needed to maintain full control of the network is determined by the 'maximum matching’ in the network, that is, the maximum set of links that do not share start or end nodes. They tried[1] to develop an analytical framework, based on the in-out degree distribution, which predicts for scale-free and Erdős–Rényi Graphs. It is however notable, that their formulation[1] would predict same values of for a chain graph and for a weak densely connected graph. Obviously, both these graphs have very different in and out degree distributions. A recent unpublished work,[2] questions whether degree, which is a purely local measure in networks, would completely describe controllability and whether even slightly distant nodes would have no role in deciding network controllability. Indeed, for many real-word networks, namely, food webs, neuronal and metabolic networks, the mismatch in values of and calculated by Liu et al.[1] is notable. It is obvious that if controllability is decided mainly by degree, why are and so different for many real world networks? They argued [1] (arXiv:1203.5161v1), that this might be due to the effect of degree correlations. However, it has been shown[2] that network controllability can be altered only by using betweenness centrality and closeness centrality, without using degree (graph theory) or degree correlations at all.
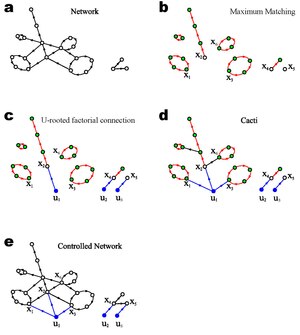
Structural Controllability
The concept of the structural properties was first introduced by Lin (1974)[3] and then extended by Shields and Pearson (1976)[4] and alternatively derived by Glover and Silverman (1976).[5] The main question is whether the lack of controllability or observability are generic with respect to the variable system parameters. In the framework of structural control the system parameters are either independent free variables or fixed zeros. This is consistent for models of physical systems since parameter values are never known exactly, with the exception of zero values which express the absence of interactions or connections.
Maximum Matching
In graph theory, a matching is a set of edges without common vertices. Liu et al.[1] extended this definition to directed graph, where a matching is a set of directed edges that do not share start or end vertices. It is easy to check that a matching of a directed graph composes of a set of vertex-disjoint simple paths and cycles. The maximum matching of a directed network can be efficiently calculated by working in the bipartite representation using the classical Hopcroft–Karp algorithm, which runs in O(E√N) time in the worst case. For undirected graph, analytical solutions of the size and number of maximum matchings have been studied using the cavity method developed in statistical physics.[6] Liu et al.[1] extended the calculations for directed graph.
By calculating the maximum matchings of a wide range of real networks, Liu et al.[1] asserted that the number of driver nodes is determined mainly by the networks degree distribution . They also calculated the average number of driver nodes for a network ensemble with arbitrary degree distribution using the cavity method. It is interesting that for a chain graph and a weak densely connected graph, both of which have very different in and out degree distributions; the formulation of Liu et al.[1] would predict same values of . Also, for many real-word networks, namely, food webs, neuronal and metabolic networks, the mismatch in values of and calculated by Liu et al.[1] is notable. If controllability is decided purely by degree, why are and so different for many real world networks? It remains open to scrutiny whether control robustness" in networks is influenced more by using betweenness centrality and closeness centrality[2] over degree (graph theory) based metrics.
While sparser graphs are more difficult to control,[1][2] it would obviously be interesting to find whether betweenness centrality and closeness centrality[2] or degree heterogeneity[1] plays a more important role in deciding controllability of sparse graphs with almost similar degree distributions.
Future Directions
This recent immense activity inspires hope of new breakthroughs in structural controllability of complex networks.
See also
References
- ↑ 1.00 1.01 1.02 1.03 1.04 1.05 1.06 1.07 1.08 1.09 1.10 1.11 1.12 Y.-Y. Liu, J.-J. Slotine, A.-L. Barabási, Nature 473 (2011).
- ↑ 2.0 2.1 2.2 2.3 2.4 SJ Banerjee and S Roy, ARXIV:1209.3737
- ↑ 3.0 3.1 C.-T. Lin, IEEE Trans. Auto. Contr. 19 (1974).
- ↑ R. W. Shields and J. B. Pearson, IEEE Trans. Auto. Contr. 21 (1976).
- ↑ K. Glover and L. M. Silverman, IEEE Trans. Auto. Contr. 21 (1976).
- ↑ L. Zdeborova and M. Mezard, J. Stat. Mech. 05 (2006).