Proper motion: Difference between revisions
No edit summary |
en>Julia W m Reverted edits by 99.182.210.16 (talk) to last version by IndividusObservantis |
||
Line 1: | Line 1: | ||
{{other uses|Optimization (disambiguation)}} | |||
[[File:MaximumParaboloid.png|right|thumb|Graph of a [[paraboloid]] given by f(''x'', ''y'') = −(''x''² + ''y''²) + 4. The global [[maximum (mathematics)|maximum]] at (0, 0, 4) is indicated by a red dot.]] | |||
In [[mathematics]], [[computer science]], or [[management science]], '''mathematical optimization''' (alternatively, '''optimization''' or '''mathematical programming''') is the selection of a best element (with regard to some criteria) from some set of available alternatives.<ref>"[http://glossary.computing.society.informs.org/index.php?page=nature.html The Nature of Mathematical Programming]," ''Mathematical Programming Glossary'', INFORMS Computing Society.</ref> | |||
In the simplest case, an [[optimization problem]] consists of [[maxima and minima|maximizing or minimizing]] a [[Function of a real variable|real function]] by systematically choosing [[Argument of a function|input]] values from within an allowed set and computing the [[Value (mathematics)|value]] of the function. The generalization of optimization theory and techniques to other formulations comprises a large area of [[applied mathematics]]. More generally, optimization includes finding "best available" values of some objective function given a defined [[domain of a function|domain]], including a variety of different types of objective functions and different types of domains. | |||
== Optimization problems == | |||
{{main|Optimization problem}} | |||
An optimization problem can be represented in the following way: | |||
:''Given:'' a [[function (mathematics)|function]] ''f'' : ''A'' <math>\to</math> '''R''' from some [[Set (mathematics)|set]] ''A'' to the [[real number]]s | |||
:''Sought:'' an element ''x''<sub>0</sub> in ''A'' such that ''f''(''x''<sub>0</sub>) ≤ ''f''(''x'') for all ''x'' in ''A'' ("minimization") or such that ''f''(''x''<sub>0</sub>) ≥ ''f''(''x'') for all ''x'' in ''A'' ("maximization"). | |||
Such a formulation is called an '''[[optimization problem]]''' or a '''mathematical programming problem''' (a term not directly related to [[computer programming]], but still in use for example in [[linear programming]] – see [[#History|History]] below). Many real-world and theoretical problems may be modeled in this general framework. Problems formulated using this technique in the fields of [[physics]] and [[computer vision]] may refer to the technique as '''energy minimization''', speaking of the value of the function ''f'' as representing the energy of the [[system]] being [[Mathematical model|modeled]]. | |||
Typically, ''A'' is some [[subset]] of the [[Euclidean space]] '''R'''<sup>''n''</sup>, often specified by a set of ''[[constraint (mathematics)|constraint]]s'', equalities or inequalities that the members of ''A'' have to satisfy. The [[domain (mathematics)|domain]] ''A'' of ''f'' is called the ''search space'' or the ''choice set'', | |||
while the elements of ''A'' are called ''[[candidate solution]]s'' or ''feasible solutions''. | |||
The function ''f'' is called, variously, an '''objective function''', a '''[[loss function]]''' or '''cost function''' (minimization),<ref>W. Erwin Diewert (2008). "cost functions," ''The New Palgrave Dictionary of Economics'', 2nd Edition [http://www.dictionaryofeconomics.com/article?id=pde2008_C000390&edition=current&q= Contents].</ref> '''indirect utility function''' (minimization),<ref>[[Peter Kenneth Newmand|Peter Newman]] (2008). "indirect utility function," ''The New Palgrave Dictionary of Economics'', 2nd Edition. [http://www.dictionaryofeconomics.com/article?id=pde2008_I000066&edition= Contents.]</ref> a '''utility function''' (maximization), a '''fitness function''' (maximization), or, in certain fields, an '''energy function''', or '''energy [[functional (mathematics)|functional]]'''. A feasible solution that minimizes (or maximizes, if that is the goal) the objective function is called an ''optimal solution''. | |||
By convention, the standard form of an optimization problem is stated in terms of minimization. Generally, unless both the objective function and the [[feasible region]] are [[convex]] in a minimization problem, there may be several local minima, where a ''local minimum'' x<sup>*</sup> is defined as a point for which there exists some δ > 0 so that for all x such that | |||
:<math>\|\mathbf{x}-\mathbf{x}^*\|\leq\delta;\,</math> | |||
the expression | |||
:<math>f(\mathbf{x}^*)\leq f(\mathbf{x})</math> | |||
holds; that is to say, on some region around x<sup>*</sup> all of the function values are greater than or equal to the value at that point. Local maxima are defined similarly. | |||
A large number of algorithms proposed for solving non-convex problems – including the majority of commercially available solvers – are not capable of making a distinction between local optimal solutions and rigorous optimal solutions, and will treat the former as actual solutions to the original problem. The branch of [[applied mathematics]] and [[numerical analysis]] that is concerned with the development of deterministic algorithms that are capable of guaranteeing convergence in finite time to the actual optimal solution of a non-convex problem is called [[global optimization]]. | |||
== Notation == | |||
Optimization problems are often expressed with special notation. Here are some examples. | |||
=== Minimum and maximum value of a function === | |||
Consider the following notation: | |||
:<math>\min_{x\in\mathbb R}\; (x^2 + 1)</math> | |||
This denotes the minimum [[Value (mathematics)|value]] of the objective function <math>x^2 + 1</math>, when choosing ''x'' from the set of [[real number]]s <math>\mathbb R</math>. The minimum value in this case is <math>1</math>, occurring at <math>x = 0</math>. | |||
Similarly, the notation | |||
:<math>\max_{x\in\mathbb R}\; 2x</math> | |||
asks for the maximum value of the objective function 2''x'', where ''x'' may be any real number. In this case, there is no such maximum as the objective function is unbounded, so the answer is "[[infinity]]" or "undefined". | |||
=== Optimal input arguments === | |||
{{Main|Arg max}} | |||
Consider the following notation: | |||
:<math>\underset{x\in(-\infty,-1]}{\operatorname{arg\,min}} \; x^2 + 1,</math> | |||
or equivalently | |||
:<math>\underset{x}{\operatorname{arg\,min}} \; x^2 + 1, \; \text{subject to:} \; x\in(-\infty,-1].</math> | |||
This represents the value (or values) of the [[Argument of a function|argument]] ''x'' in the [[interval (mathematics)|interval]] <math>(-\infty,-1]</math> that minimizes (or minimize) the objective function ''x''<sup>2</sup> + 1 (the actual minimum value of that function is not what the problem asks for). In this case, the answer is ''x'' = -1, since ''x'' = 0 is infeasible, i.e. does not belong to the [[feasible set]]. | |||
Similarly, | |||
:<math>\underset{x\in[-5,5], \; y\in\mathbb R}{\operatorname{arg\,max}} \; x\cos(y),</math> | |||
or equivalently | |||
:<math>\underset{x, \; y}{\operatorname{arg\,max}} \; x\cos(y), \; \text{subject to:} \; x\in[-5,5], \; y\in\mathbb R,</math> | |||
represents the <math>(x,y)</math> pair (or pairs) that maximizes (or maximize) the value of the objective function <math>x\cos(y)</math>, with the added constraint that ''x'' lie in the interval <math>[-5,5]</math> (again, the actual maximum value of the expression does not matter). In this case, the solutions are the pairs of the form (5, 2k[[Pi|π]]) and (−5,(2k+1)π), where ''k'' ranges over all [[integer]]s. | |||
'''Arg min''' and '''arg max''' are sometimes also written '''argmin''' and '''argmax''', and stand for '''argument of the minimum''' and '''argument of the maximum'''. | |||
== History == | |||
[[Pierre de Fermat|Fermat]] and [[Joseph Louis Lagrange|Lagrange]] found calculus-based formulas for identifying optima, while [[Isaac Newton|Newton]] and [[Carl Friedrich Gauss|Gauss]] proposed iterative methods for moving towards an optimum. Historically, the first term for optimization was "[[linear programming]]", which was due to [[George Dantzig|George B. Dantzig]], although much of the theory had been introduced by [[Leonid Kantorovich]] in 1939. Dantzig published the [[Simplex algorithm]] in 1947, and [[John von Neumann]] developed the theory of [[Linear programming#Duality|duality]] in the same year. | |||
The term, ''programming'', in this context does not refer to [[computer programming]]. Rather, the term comes from the use of ''program'' by the United States military to refer to proposed training and [[logistics]] schedules, which were the problems Dantzig studied at that time. | |||
Later important researchers in mathematical optimization include the following: | |||
{{col-begin}} | |||
{{col-2}} | |||
* [[Richard Bellman]] | |||
* [[Roger Fletcher (mathematician)|Roger Fletcher]] | |||
* [[Ronald A. Howard]] | |||
* [[Narendra Karmarkar]] | |||
* [[William Karush]] | |||
* [[Leonid Khachiyan]] | |||
* [[Bernard Koopman]] | |||
* [[Harold Kuhn]] | |||
* [[Joseph Louis Lagrange]] | |||
* [[László Lovász]] | |||
{{col-2}} | |||
* [[Arkadi Nemirovski]] | |||
* [[Yurii Nesterov]] | |||
* [[Boris Polyak]] | |||
* [[Lev Pontryagin]] | |||
* [[James Renegar]] | |||
* [[R. Tyrrell Rockafellar]] | |||
* [[Cornelis Roos]] | |||
* [[Naum Z. Shor]] | |||
* [[Michael J. Todd (mathematician)|Michael J. Todd]] | |||
* [[Albert W. Tucker|Albert Tucker]] | |||
{{col-end}} | |||
<!--In fact, some mathematical programming work had been done previously... (anyone? - Gauss did some stuff here), Gauss developed the method of least squares, which is an optimization method. --> | |||
== Major subfields == | |||
* [[Convex programming]] studies the case when the objective function is [[convex function|convex]] (minimization) or [[Concave function|concave]] (maximization) and the constraint set is [[convex set|convex]]. This can be viewed as a particular case of nonlinear programming or as generalization of linear or convex quadratic programming. | |||
** [[Linear programming]] (LP), a type of convex programming, studies the case in which the objective function ''f'' is linear and the set of constraints is specified using only linear equalities and inequalities. Such a set is called a [[polyhedron]] or a [[polytope]] if it is [[Bounded set|bounded]]. | |||
** [[Second order cone programming]] (SOCP) is a convex program, and includes certain types of quadratic programs. | |||
** [[Semidefinite programming]] (SDP) is a subfield of convex optimization where the underlying variables are [[semidefinite]] [[matrix (mathematics)|matrices]]. It is generalization of linear and convex quadratic programming. | |||
** [[Conic programming]] is a general form of convex programming. LP, SOCP and SDP can all be viewed as conic programs with the appropriate type of cone. | |||
** [[Geometric programming]] is a technique whereby objective and inequality constraints expressed as [[posynomials]] and equality constraints as [[monomials]] can be transformed into a convex program. | |||
* [[Integer programming]] studies linear programs in which some or all variables are constrained to take on [[integer]] values. This is not convex, and in general much more difficult than regular linear programming. | |||
* [[Quadratic programming]] allows the objective function to have quadratic terms, while the feasible set must be specified with linear equalities and inequalities. For specific forms of the quadratic term, this is a type of convex programming. | |||
* [[Fractional programming]] studies optimization of ratios of two nonlinear functions. The special class of concave fractional programs can be transformed to a convex optimization problem. | |||
* [[Nonlinear programming]] studies the general case in which the objective function or the constraints or both contain nonlinear parts. This may or may not be a convex program. In general, whether the program is convex affects the difficulty of solving it. | |||
* [[Stochastic programming]] studies the case in which some of the constraints or parameters depend on [[random variable]]s. | |||
* [[Robust optimization|Robust programming]] is, like stochastic programming, an attempt to capture uncertainty in the data underlying the optimization problem. This is not done through the use of random variables, but instead, the problem is solved taking into account inaccuracies in the input data. | |||
* [[Combinatorial optimization]] is concerned with problems where the set of feasible solutions is discrete or can be reduced to a [[discrete mathematics|discrete]] one. | |||
* [[Stochastic optimization]] for use with random (noisy) function measurements or random inputs in the search process. | |||
* [[Infinite-dimensional optimization]] studies the case when the set of feasible solutions is a subset of an infinite-[[dimension]]al space, such as a space of functions. | |||
* [[Heuristic (computer science)|Heuristics]] and [[metaheuristic]]s make few or no assumptions about the problem being optimized. Usually, heuristics do not guarantee that any optimal solution need be found. On the other hand, heuristics are used to find approximate solutions for many complicated optimization problems. | |||
* [[Constraint satisfaction]] studies the case in which the objective function ''f'' is constant (this is used in [[artificial intelligence]], particularly in [[automated reasoning]]). | |||
** [[Constraint programming]]. | |||
* Disjunctive programming is used where at least one constraint must be satisfied but not all. It is of particular use in scheduling. | |||
In a number of subfields, the techniques are designed primarily for optimization in dynamic contexts (that is, decision making over time): | |||
* [[Calculus of variations]] seeks to optimize an objective defined over many points in time, by considering how the objective function changes if there is a small change in the choice path. | |||
* [[Optimal control]] theory is a generalization of the calculus of variations. | |||
* [[Dynamic programming]] studies the case in which the optimization strategy is based on splitting the problem into smaller subproblems. The equation that describes the relationship between these subproblems is called the [[Bellman equation]]. | |||
* [[Mathematical programming with equilibrium constraints]] is where the constraints include [[variational inequalities]] or [[complementarity theory|complementarities]]. | |||
=== Multi-objective optimization === | |||
{{Main|Multi-objective optimization}} | |||
Adding more than one objective to an optimization problem adds complexity. For example, to optimize a structural design, one would want a design that is both light and rigid. Because these two objectives conflict, a trade-off exists. There will be one lightest design, one stiffest design, and an infinite number of designs that are some compromise of weight and stiffness. The set of trade-off designs that cannot be improved upon according to one criterion without hurting another criterion is known as the [[Pareto set]]. The curve created plotting weight against stiffness of the best designs is known as the [[Pareto frontier]]. | |||
A design is judged to be "Pareto optimal" (equivalently, "Pareto efficient" or in the Pareto set) if it is not dominated by any other design: If it is worse than another design in some respects and no better in any respect, then it is dominated and is not Pareto optimal. | |||
The choice among "Pareto optimal" solutions to determine the "favorite solution" is delegated to the decision maker. In other words, defining the problem as multiobjective optimization signals that some information is missing: desirable objectives are given but not their detailed combination. In some cases, the missing information can be derived by interactive sessions with the decision maker. | |||
Multi-objective optimization problems have been generalized further to [[vector optimization]] problems where the (partial) ordering is no longer given by the Pareto ordering. | |||
=== Multi-modal optimization === | |||
Optimization problems are often multi-modal; that is, they possess multiple good solutions. They could all be globally good (same cost function value) or there could be a mix of globally good and locally good solutions. Obtaining all (or at least some of) the multiple solutions is the goal of a multi-modal optimizer. | |||
Classical optimization techniques due to their iterative approach do not perform satisfactorily when they are used to obtain multiple solutions, since it is not guaranteed that different solutions will be obtained even with different starting points in multiple runs of the algorithm. [[Evolutionary Algorithm]]s are however a very popular approach to obtain multiple solutions in a multi-modal optimization task. | |||
== Classification of critical points and extrema == | |||
=== Feasibility problem === | |||
The '''[[satisfiability problem]]''', also called the '''feasibility problem''', is just the problem of finding any [[feasible solution]] at all without regard to objective value. This can be regarded as the special case of mathematical optimization where the objective value is the same for every solution, and thus any solution is optimal. | |||
Many optimization algorithms need to start from a feasible point. One way to obtain such a point is to [[Relaxation (approximation)|relax]] the feasibility conditions using a [[slack variable]]; with enough slack, any starting point is feasible. Then, minimize that slack variable until slack is null or negative. | |||
=== Existence === | |||
The [[extreme value theorem]] of [[Karl Weierstrass]] states that a continuous real-valued function on a compact set attains its maximum and minimum value. More generally, a lower semi-continuous function on a compact set attains its minimum; an upper semi-continuous function on a compact set attains its maximum. | |||
=== Necessary conditions for optimality === | |||
[[Fermat's theorem (stationary points)|One of Fermat's theorems]] states that optima of unconstrained problems are found at [[stationary point]]s, where the first derivative or the gradient of the objective function is zero (see [[first derivative test]]). More generally, they may be found at [[Critical point (mathematics)|critical points]], where the first derivative or gradient of the objective function is zero or is undefined, or on the boundary of the choice set. An equation (or set of equations) stating that the first derivative(s) equal(s) zero at an interior optimum is called a 'first-order condition' or a set of first-order conditions. | |||
Optima of equality-constrained problems can be found by the [[Lagrange multiplier]] method. The optima of problems with equality and/or inequality constraints can be found using the '[[Karush–Kuhn–Tucker conditions]]'. | |||
=== Sufficient conditions for optimality === | |||
While the first derivative test identifies points that might be extrema, this test does not distinguish a point that is a minimum from one that is a maximum or one that is neither. When the objective function is twice differentiable, these cases can be distinguished by checking the second derivative or the matrix of second derivatives (called the [[Hessian matrix]]) in unconstrained problems, or the matrix of second derivatives of the objective function and the constraints called the [[Hessian matrix#Bordered Hessian|bordered Hessian]] in constrained problems. The conditions that distinguish maxima, or minima, from other stationary points are called 'second-order conditions' (see '[[Second derivative test]]'). If a candidate solution satisfies the first-order conditions, then satisfaction of the second-order conditions as well is sufficient to establish at least local optimality. | |||
=== Sensitivity and continuity of optima === | |||
The [[envelope theorem]] describes how the value of an optimal solution changes when an underlying [[parameter]] changes. The process of computing this change is called [[comparative statics]]. | |||
The [[maximum theorem]] of [[Claude Berge]] (1963) describes the continuity of an optimal solution as a function of underlying parameters. | |||
===Calculus of optimization=== | |||
{{Main|Karush–Kuhn–Tucker conditions}} | |||
{{See also|Critical point (mathematics)|Differential calculus|Gradient|Hessian matrix|Positive definite matrix|Lipschitz continuity|Rademacher's theorem|Convex function|Convex analysis}} | |||
For unconstrained problems with twice-differentiable functions, some [[critical point (mathematics)|critical point]]s can be found by finding the points where the [[gradient]] of the objective function is zero (that is, the stationary points). More generally, a zero [[subgradient]] certifies that a local minimum has been found for [[convex optimization|minimization problems with convex]] [[convex function|function]]s and other [[Rademacher's theorem|locally]] [[Lipschitz function]]s. | |||
Further, critical points can be classified using the [[positive definite matrix|definiteness]] of the [[Hessian matrix]]: If the Hessian is ''positive'' definite at a critical point, then the point is a local minimum; if the Hessian matrix is negative definite, then the point is a local maximum; finally, if indefinite, then the point is some kind of [[saddle point]]. | |||
Constrained problems can often be transformed into unconstrained problems with the help of [[Lagrange multiplier]]s. [[Lagrangian relaxation]] can also provide approximate solutions to difficult constrained problems. | |||
When the objective function is [[Convex function|convex]], then any local minimum will also be a global minimum. There exist efficient numerical techniques for minimizing convex functions, such as [[interior-point method]]s. | |||
== Computational optimization techniques == | |||
To solve problems, researchers may use [[algorithm]]s that terminate in a finite number of steps, or [[iterative method]]s that converge to a solution (on some specified class of problems), or [[heuristic algorithm|heuristics]] that may provide approximate solutions to some problems (although their iterates need not converge). | |||
===Optimization algorithms=== | |||
* [[Simplex algorithm]] of [[George Dantzig]], designed for [[linear programming]]. | |||
* Extensions of the simplex algorithm, designed for [[quadratic programming]] and for [[linear-fractional programming]]. | |||
* Variants of the simplex algorithm that are especially suited for [[flow network|network optimization]]. | |||
* [[Combinatorial optimization|Combinatorial algorithm]]s | |||
===Iterative methods=== | |||
{{Main|Iterative method}} | |||
{{See also|Newton's method|Quasi-Newton method|Finite difference|Approximation theory|Numerical analysis}} | |||
The [[iterative methods]] used to solve problems of [[nonlinear programming]] differ according to whether they [[subroutine|evaluate]] Hessians, gradients, or only function values. While evaluating Hessians (H) and gradients (G) improves the rate of convergence, for functions for which these quantities exist and vary sufficiently smoothly, such evaluations increase the [[computational complexity]] (or computational cost) of each iteration. In some cases, the computational complexity may be excessively high. | |||
One major criterion for optimizers is just the number of required function evaluations as this often is already a large computational effort, usually much more effort than within the optimizer itself, which mainly has to operate over the N variables. | |||
The derivatives provide detailed information for such optimizers, but are even harder to calculate, e.g. approximating the gradient takes at least N+1 function evaluations. For approximations of the 2nd derivatives (collected in the Hessian matrix) the number of function evaluations is in the order of N². Newton's method requires the 2nd order derivates, so for each iteration the number of function calls is in the order of N², but for a simpler pure gradient optimizer it is only N. However, gradient optimizers need usually more iterations than Newton's algorithm. Which one is best with respect to the number of function calls depends on the problem itself. | |||
* Methods that evaluate Hessians (or approximate Hessians, using [[finite difference]]s): | |||
** [[Newton's method in optimization|Newton's method]] | |||
*** [[Sequential quadratic programming]]: A Newton-based method for small-medium scale ''constrained'' problems. Some versions can handle large-dimensional problems. | |||
* Methods that evaluate gradients or approximate gradients using finite differences (or even subgradients): | |||
** [[Quasi-Newton method]]s: Iterative methods for medium-large problems (e.g. N<1000). | |||
** [[Conjugate gradient method]]s: [[Iterative method]]s for large problems. (In theory, these methods terminate in a finite number of steps with quadratic objective functions, but this finite termination is not observed in practice on finite–precision computers.) | |||
** [[Interior point methods]]: This is a large class of methods for constrained optimization. Some interior-point methods use only (sub)gradient information, and others of which require the evaluation of Hessians. | |||
** [[Gradient descent]] (alternatively, "steepest descent" or "steepest ascent"): A (slow) method of historical and theoretical interest, which has had renewed interest for finding approximate solutions of enormous problems. | |||
** [[Subgradient method]]s - An iterative method for large [[Rademacher's theorem|locally]] [[Lipschitz continuity|Lipschitz function]]s using [[subgradient|generalized gradient]]s. Following Boris T. Polyak, subgradient–projection methods are similar to conjugate–gradient methods. | |||
** Bundle method of descent: An iterative method for small–medium sized problems with locally Lipschitz functions, particularly for [[convex optimization|convex minimization]] problems. (Similar to conjugate gradient methods) | |||
** [[Ellipsoid method]]: An iterative method for small problems with [[quasiconvex function|quasiconvex]] objective functions and of great theoretical interest, particularly in establishing the polynomial time complexity of some combinatorial optimization problems. It has similarities with Quasi-Newton methods. | |||
** [[Frank–Wolfe algorithm|Reduced gradient method (Frank–Wolfe)]] for approximate minimization of specially structured problems with [[linear constraints]], especially with traffic networks. For general unconstrained problems, this method reduces to the gradient method, which is regarded as obsolete (for almost all problems). | |||
** [[Simultaneous perturbation stochastic approximation]] (SPSA) method for stochastic optimization; uses random (efficient) gradient approximation. | |||
* Methods that evaluate only function values: If a problem is continuously differentiable, then gradients can be approximated using finite differences, in which case a gradient-based method can be used. | |||
** [[Interpolation]] methods | |||
** [[Pattern search (optimization)|Pattern search]] methods, which have better convergence properties than the [[Nelder–Mead method|Nelder–Mead heuristic (with simplices)]], which is listed below. | |||
====Global convergence==== | |||
More generally, if the objective function is not a quadratic function, then many optimization methods use other methods to ensure that some subsequence of iterations converges to an optimal solution. The first and still popular method for ensuring convergence relies on [[line search]]es, which optimize a function along one dimension. A second and increasingly popular method for ensuring convergence uses [[trust region]]s. Both line searches and trust regions are used in modern methods of [[subgradient method|non-differentiable optimization]]. Usually a global optimizer is much slower than advanced local optimizers (such as [[BFGS method|BFGS]]), so often an efficient global optimizer can be constructed by starting the local optimizer from different starting points. | |||
===Heuristics=== | |||
{{Main|Heuristic algorithm}} | |||
Besides (finitely terminating) [[algorithm]]s and (convergent) [[iterative method]]s, there are [[heuristic algorithm|heuristics]] that can provide approximate solutions to some optimization problems: | |||
* [[Memetic algorithm]] | |||
* [[Differential evolution]] | |||
* [[Differential Search algorithm]] <ref>{{cite journal|last=Civicioglu|first=P.|title=Transforming geocentric cartesian coordinates to geodetic coordinates by using differential search algorithm|journal=Computers & Geosciences|year=2012|volume=46|pages=229–247|doi=10.1016/j.cageo.2011.12.011}}</ref> Matlab code-link has been provided in Çivicioglu, P.,(2012). | |||
* [[Dynamic relaxation]] | |||
* [[Genetic algorithms]] | |||
* [[Hill climbing]] | |||
* [[Nelder-Mead method|Nelder-Mead simplicial heuristic]]: A popular heuristic for approximate minimization (without calling gradients) | |||
* [[Particle swarm optimization]] | |||
* [[Artificial bee colony optimization]] | |||
* [[Simulated annealing]] | |||
* [[Tabu search]] | |||
* [[Reactive Search Optimization|Reactive Search Optimization (RSO)]]<ref> | |||
{{cite book | |||
|title=Reactive Search and Intelligent Optimization | |||
|last=Battiti | |||
|first=Roberto | |||
|authorlink= | |||
|coauthors=Mauro Brunato; Franco Mascia | |||
|url =http://reactive-search.org/thebook | |||
|year=2008 | |||
|publisher=[[Springer Verlag]] | |||
|location= | |||
|isbn=978-0-387-09623-0 | |||
}} | |||
</ref> implemented in [[LIONsolver]] | |||
== Applications == | |||
===Mechanics and engineering=== | |||
Problems in [[rigid body dynamics]] (in particular articulated rigid body dynamics) often require mathematical programming techniques, since you can view rigid body dynamics as attempting to solve an [[ordinary differential equation]] on a constraint manifold; the constraints are various nonlinear geometric constraints such as "these two points must always coincide", "this surface must not penetrate any other", or "this point must always lie somewhere on this curve". Also, the problem of computing contact forces can be done by solving a [[linear complementarity problem]], which can also be viewed as a QP (quadratic programming) problem. | |||
Many design problems can also be expressed as optimization programs. This application is called design optimization. One subset is the [[engineering optimization]], and another recent and growing subset of this field is [[multidisciplinary design optimization]], which, while useful in many problems, has in particular been applied to [[aerospace engineering]] problems. | |||
===Economics=== | |||
[[Economics]] is closely enough linked to optimization of [[agent (economics)|agents]] that an influential definition relatedly describes economics ''qua'' science as the "study of human behavior as a relationship between ends and [[scarce]] means" with alternative uses.<ref>[[Lionel Robbins]] (1935, 2nd ed.) ''[[An Essay on the Nature and Significance of Economic Science#Major propositions|An Essay on the Nature and Significance of Economic Science]]'', Macmillan, p. 16.</ref> Modern optimization theory includes traditional optimization theory but also overlaps with [[game theory]] and the study of economic [[equilibrium (economics)|equilibria]]. The ''[[Journal of Economic Literature]]'' [[JEL classification codes|codes]] classify mathematical programming, optimization techniques, and related topics under [[JEL classification codes#Mathematical and quantitative methods JEL: C Subcategories|JEL:C61-C63]]. | |||
In microeconomics, the [[utility maximization problem]] and its [[dual problem]], the [[expenditure minimization problem]], are economic optimization problems. Insofar as they behave consistently, [[consumer]]s are assumed to maximize their [[utility]], while [[firm]]s are usually assumed to maximize their [[Profit (economics)|profit]]. Also, agents are often modeled as being [[Risk aversion|risk-averse]], thereby preferring to avoid risk. [[Asset pricing|Asset prices]] are also modeled using optimization theory, though the underlying mathematics relies on optimizing [[stochastic process]]es rather than on static optimization. [[Trade]] theory also uses optimization to explain trade patterns between nations. The optimization of [[Portfolio (finance)|market portfolios]] is an example of multi-objective optimization in economics. | |||
Since the 1970s, economists have modeled dynamic decisions over time using [[control theory]]. For example, microeconomists use [[dynamic programming|dynamic]] [[search theory|search model]]s to study [[labor economics|labor-market behavior]].<ref>[[Avinash Dixit|A. K. Dixit]] ([1976] 1990). ''Optimization in Economic Theory'', 2nd ed., Oxford. [http://books.google.com/books?id=dHrsHz0VocUC&pg=find&pg=PA194=false#v=onepage&q&f=false Description] and contents [http://books.google.com/books?id=dHrsHz0VocUC&pg=PR7&lpg=PR6&dq=false&lr=#v=onepage&q=false&f=false preview].</ref> A crucial distinction is between deterministic and stochastic models.<ref>A.G. Malliaris (2008). "stochastic optimal control," ''The New Palgrave Dictionary of Economics'', 2nd Edition. [http://www.dictionaryofeconomics.com/article?id=pde2008_S000269&edition=&field=keyword&q=Taylor's%20th&topicid=&result_number=1 Abstract].</ref> [[Macroeconomics|Macroeconomists]] build [[dynamic stochastic general equilibrium|dynamic stochastic general equilibrium (DSGE)]] models that describe the dynamics of the whole economy as the result of the interdependent optimizing decisions of workers, consumers, investors, and governments.<ref>[[Julio Rotemberg]] and [[Michael Woodford (economist)|Michael Woodford]] (1997), "An Optimization-based Econometric Framework for the Evaluation of Monetary Policy.''NBER Macroeconomics Annual'', 12, pp. [http://people.hbs.edu/jrotemberg/PublishedArticles/OptimizBasedEconometric_97.pdf 297-346.]</ref><ref>From ''[[The New Palgrave Dictionary of Economics]]'' (2008), 2nd Edition with Abstract links:<br/> • "[http://www.dictionaryofeconomics.com/article?id=pde2008_N000148&edition=current&q=optimization&topicid=&result_number=1 numerical optimization methods in economics]" by Karl Schmedders<br/> • "[http://www.dictionaryofeconomics.com/article?id=pde2008_C000348&edition=current&q=optimization&topicid=&result_number=4 convex programming]" by [[Lawrence E. Blume]]<br/> • "[http://www.dictionaryofeconomics.com/article?id=pde2008_A000133&edition=current&q=optimization&topicid=&result_number=20 Arrow–Debreu model of general equilibrium]" by [[John Geanakoplos]].</ref> | |||
===Operations research=== | |||
Another field that uses optimization techniques extensively is [[operations research]].<ref>{{cite web|title=New force on the political scene: the Seophonisten|url=http://www.seophonist-wahl.de/|publisher=http://www.seophonist-wahl.de|accessdate=14 September 2013}}</ref> Operations research also uses stochastic modeling and simulation to support improved decision-making. Increasingly, operations research uses [[stochastic programming]] to model dynamic decisions that adapt to events; such problems can be solved with large-scale optimization and [[stochastic optimization]] methods. | |||
===Control engineering=== | |||
Mathematical optimization is used in much modern controller design. High-level controllers such as [[Model predictive control]] (MPC) or Real-Time Optimization (RTO) employ mathematical optimization. These algorithms run online and repeatedly determine values for decision variables, such as choke openings in a process plant, by iteratively solving a mathematical optimization problem including constraints and a model of the system to be controlled. | |||
===Petroleum engineering=== | |||
An important application of optimization is constructing computational models of oil reservoirs.<ref>Gharib Shirangi, M., History matching production data and uncertainty assessment with an efficient TSVD parameterization algorithm, Journal of Petroleum Science and Engineering, http://www.sciencedirect.com/science/article/pii/S0920410513003227</ref> | |||
== Solvers == | |||
{{main|List of optimization software}} | |||
== See also == | |||
{{multicol}} | |||
*[[Curve fitting]] | |||
*[[Brachistochrone]] | |||
*[[Goal programming]] | |||
*[[List of publications in mathematics#Optimization|Important publications in optimization]] | |||
{{multicol-break}} | |||
*[[Least squares]] | |||
*[[Mathematical Optimization Society]] (formerly Mathematical Programming Society) | |||
{{multicol-break}} | |||
*[[:Category:Mathematical optimization software|Mathematical optimization software]] | |||
*[[:Category:Optimization algorithms and methods|Mathematical optimization algorithms]] | |||
*[[Process optimization]] | |||
*[[Variational calculus]] | |||
{{multicol-end}} | |||
==Notes== | |||
{{Reflist}} | |||
==Further reading== | |||
===Comprehensive=== | |||
====Undergraduate level==== | |||
*{{cite book|title=Applied mathematical programming|last1=Bradley|first1=S.|last2=Hax|first2=A.|last3=Magnanti|first3=T.|authorlink3=Thomas Magnanti|year=1977|publisher=Addison Wesley}} | |||
* {{cite book|title=Optimization in operations research|first=Ronald L.|last=Rardin|year=1997|publisher=Prentice Hall|copyright=1998|pages=919|isbn=0-02-398415-5}} | |||
* {{cite book|last=Strang|first=Gilbert|authorlink=Gilbert Strang|title=Introduction to applied mathematics|publisher=Wellesley-Cambridge Press (Strang's publishing company)|location=Wellesley, MA|year=1986|pages=xii+758|isbn=0-9614088-0-4|url=http://www.wellesleycambridge.com/tocs/toc-appl|mr=870634|ref=harv}} | |||
====Graduate level==== | |||
* {{cite book|last=Magnanti|first=Thomas L.|authorlink=Thomas L. Magnanti|year=1989|chapter=Twenty years of mathematical programming|pages=163–227|editor-first=Bernard|editor-last=Cornet|editor2-first=Henry|editor2-last=Tulkens|title=Contributions to Operations Research and Economics: The twentieth anniversary of CORE (Papers from the symposium held in Louvain-la-Neuve, January 1987)|publisher=MIT Press|location=Cambridge, MA|isbn=0-262-03149-3|mr=1104662|ref=harv}} | |||
* {{cite book|last=Minoux|first=M.|authorlink=Michel Minoux|title=Mathematical programming: Theory and algorithms|note=With a foreword by Egon Balas|edition=Translated by Steven Vajda from the (1983 Paris: Dunod) French|publisher=A Wiley-Interscience Publication. John Wiley & Sons, Ltd.|location=Chichester|year=1986|pages=xxviii+489|isbn=0-471-90170-9|mr=868279|ref=harv|id=(2008 Second ed., in French: ''Programmation mathématique: Théorie et algorithmes''. Editions Tec & Doc, Paris, 2008. xxx+711 pp. ISBN 978-2-7430-1000-3. |mr =2571910}} | |||
* {{cite book|title=Optimization|editor1-first=G. L.|editor1-last=Nemhauser|editor1-link=George L. Nemhauser|editor2-first=A. H. G.|editor2-last=Rinnooy Kan|editor3-first=M. J.|editor3-last=Todd|editor3-link=Michael J. Todd (mathematician)|series=Handbooks in Operations Research and Management Science|volume=1|publisher=North-Holland Publishing Co.|location=Amsterdam|year=1989|pages=xiv+709|isbn=0-444-87284-1|mr=1105099|ref=harv}} | |||
** [[J. E. Dennis, Jr.]] and [[Robert B. Schnabel]], A view of unconstrained optimization (pp. 1–72); | |||
** [[Donald Goldfarb]] and [[Michael J. Todd (mathematician)|Michael J. Todd]], Linear programming (pp. 73–170); | |||
** Philip E. Gill, Walter Murray, Michael A. Saunders, and [[Margaret H. Wright]], Constrained nonlinear programming (pp. 171–210); | |||
** [[Ravindra K. Ahuja]], [[Thomas L. Magnanti]], and [[James B. Orlin]], Network flows (pp. 211–369); | |||
** [[W. R. Pulleyblank]], Polyhedral combinatorics (pp. 371–446); | |||
** George L. Nemhauser and Laurence A. Wolsey, Integer programming (pp. 447–527); | |||
** [[Claude Lemaréchal]], Nondifferentiable optimization (pp. 529–572); | |||
** [[Roger J-B Wets]], Stochastic programming (pp. 573–629); | |||
** A. H. G. Rinnooy Kan and G. T. Timmer, Global optimization (pp. 631–662); | |||
** P. L. Yu, Multiple criteria decision making: five basic concepts (pp. 663–699). | |||
* {{cite book|last=Shapiro|first=Jeremy F.|title=Mathematical programming: Structures and algorithms|publisher=Wiley-Interscience [John Wiley & Sons]|location=New York|year=1979|pages=xvi+388|ISBN=0-471-77886-9|mr=544669|ref=harv}} | |||
* Spall, J. C. (2003), ''Introduction to Stochastic Search and Optimization: Estimation, Simulation, and Control'', Wiley, Hoboken, NJ. | |||
===Continuous optimization=== | |||
* {{cite book| author=Roger Fletcher |year=2000 | title=Practical methods of optimization | publisher=Wiley | isbn= 978-0-471-49463-8}} | |||
* {{cite book| author=Mordecai Avriel |year=2003 | title=Nonlinear Programming: Analysis and Methods | publisher=Dover Publishing| isbn= 0-486-43227-0}} | |||
* {{cite book| author=P. E. Gill, W. Murray and M. H. Wright | year=1982 | title=Practical Optimization | publisher= Emerald Publishing |isbn=978-0122839528}} | |||
* {{cite book| author=Xin-She Yang |year=2010 |title=Engineering Optimization: An Introduction with Metaheuristic Applications | publisher=Wiley |isbn=978-0470582466}} | |||
* {{cite book|last1=Bonnans|first1=J. Frédéric|last2=Gilbert|first2=J. Charles|last3=Lemaréchal|first3=Claude|last4=Sagastizábal|first4=Claudia A.|title=Numerical optimization: Theoretical and practical aspects|url=http://www.springer.com/mathematics/applications/book/978-3-540-35445-1|edition=Second revised ed. of translation of 1997 <!-- ''Optimisation numérique: Aspects théoriques et pratiques'' --> French| series=Universitext|publisher=Springer-Verlag|location=Berlin|year=2006|pages=xiv+490|isbn=3-540-35445-X|doi=10.1007/978-3-540-35447-5|mr=2265882|unused_data=<!-- authorlink3=Claude Lemaréchal -->}} | |||
* {{cite book|last1=Bonnans|first1=J. Frédéric|last2=Shapiro|first2=Alexander|title=Perturbation analysis of optimization problems|series=Springer Series in Operations Research|publisher=Springer-Verlag|location=New York|year=2000|pages=xviii+601|isbn=0-387-98705-3|mr=1756264}} | |||
* {{cite book|title=Convex Optimization|first1=Stephen P.|last1=Boyd|first2=Lieven|last2=Vandenberghe|year=2004|publisher=Cambridge University Press|isbn=978-0-521-83378-3|url=http://www.stanford.edu/~boyd/cvxbook/bv_cvxbook.pdf|format=pdf|accessdate=October 15, 2011}} | |||
* {{cite book | year=2006|url=http://www.ece.northwestern.edu/~nocedal/book/num-opt.html| title= Numerical Optimization| publisher=Springer|isbn=0-387-30303-0| author=Jorge Nocedal and Stephen J. Wright}} | |||
* {{cite book|last=[[Andrzej Piotr Ruszczyński|Ruszczyński]]|first=Andrzej|title=Nonlinear Optimization|publisher=[[Princeton University Press]]|location=Princeton, NJ|year=2006|pages=xii+454|isbn=978-0691119151 |mr=2199043}} | |||
===Combinatorial optimization=== | |||
* R. K. Ahuja, [[Thomas L. Magnanti]], and [[James B. Orlin]] (1993). ''Network Flows: Theory, Algorithms, and Applications''. Prentice-Hall, Inc. ISBN 0-13-617549-X. | |||
* [[William J. Cook]], William H. Cunningham, William R. Pulleyblank, [[Alexander Schrijver]]; ''Combinatorial Optimization''; John Wiley & Sons; 1 edition (November 12, 1997); ISBN 0-471-55894-X. | |||
* {{cite book|last1=Gondran|first1=Michel|last2=Minoux|first2=Michel|title=Graphs and algorithms|edition=Translated by Steven Vajda from the second (''Collection de la Direction des Études et Recherches d'Électricité de France'' [Collection of the Department of Studies and Research of Électricité de France], v. 37. Paris: Éditions Eyrolles 1985. xxviii+545 pp. {{MR|868083}}) French|series=Wiley-Interscience Series in Discrete Mathematics|publisher=John Wiley & Sons, Ltd.|location= Chichester|year=1984|pages=xix+650|isbn=0-471-10374-8|mr=745802|id=(Fourth ed. Collection EDF R&D. Paris: Editions Tec & Doc 2009. xxxii+784 pp.| ISBN =978-2-7430-1035-5|ref=harv| mr=2552933 }} | |||
* {{cite book | author = Eugene Lawler | authorlink = Eugene Lawler | title = Combinatorial Optimization: Networks and Matroids <!-- chapter = 4.5. Combinatorial Implications of Max-Flow Min-Cut Theorem, 4.6. Linear Programming Interpretation of Max-Flow Min-Cut Theorem -->| year = 2001 | publisher = Dover | isbn = 0-486-41453-1 <!-- pages = 117–120 -->}} | |||
*{{citation|first1 = E. L.|last1=Lawler|author1-link=Eugene Lawler|first2=J. K.|last2=Lenstra|author2-link=Jan Karel Lenstra|first3=A. H. G.|last3=Rinnooy Kan|first4=D. B.|last4=Shmoys | title = The traveling salesman problem: A guided tour of combinatorial optimization | year = 1985 | publisher = John Wiley & Sons | isbn = 0-471-90413-9}}. | |||
*[[Jon Lee (mathematician)|Jon Lee]]; ''[http://books.google.com/books?id=3pL1B7WVYnAC&printsec=frontcover&source=gbs_ge_summary_r&cad=0#v=onepage&q&f=false A First Course in Combinatorial Optimization]''; Cambridge University Press; 2004; ISBN 0-521-01012-8. | |||
*Christos H. Papadimitriou and [[Kenneth Steiglitz]] ''Combinatorial Optimization : Algorithms and Complexity''; Dover Pubns; (paperback, Unabridged edition, July 1998) ISBN 0-486-40258-4. | |||
==Journals== | |||
* [http://www.springer.com/mathematics/journal/10589 ''Computational Optimization and Applications''] | |||
* [https://www.novapublishers.com/catalog/product_info.php?products_id=6353 Journal of Computational ''Optimization in Economics and Finance''] | |||
* [http://www.journals.elsevier.com/journal-of-economic-dynamics-and-control/ ''Journal of Economic Dynamics and Control''] | |||
* [http://www.siam.org/journals/siopt.php ''SIAM Journal on Optimization'' (SIOPT)] and [http://www.siam.org/journals/siopt/policy.php Editorial Policy] | |||
* [http://www.siam.org/journals/sicon.php ''SIAM Journal on Control and Optimization'' (SICON)] and [http://www.siam.org/journals/sicon/policy.php Editorial Policy] | |||
== External links == | |||
* [http://www.coin-or.org/ COIN-OR]—Computational Infrastructure for Operations Research | |||
* [http://plato.asu.edu/guide.html Decision Tree for Optimization Software] Links to optimization source codes | |||
* [http://www.mat.univie.ac.at/%7Eneum/glopt.html Global optimization] | |||
* [http://glossary.computing.society.informs.org/ Mathematical Programming Glossary] | |||
* [http://www.mathprog.org/ Mathematical Programming Society] | |||
* [http://www-fp.mcs.anl.gov/otc/Guide/index.html NEOS Guide] currently being replaced by the [http://wiki.mcs.anl.gov/neos NEOS Wiki] | |||
* [http://www.optimization-online.org Optimization Online] A repository for optimization e-prints | |||
* [http://www2.arnes.si/%7Eljc3m2/igor/links.html Optimization Related Links] | |||
* [http://see.stanford.edu/see/courseinfo.aspx?coll=2db7ced4-39d1-4fdb-90e8-364129597c87 Convex Optimization I] EE364a: Course from Stanford University | |||
* [http://www.stanford.edu/~boyd/cvxbook Convex Optimization – Boyd and Vandenberghe] Book on Convex Optimization | |||
* [http://apmonitor.com/me575/index.php/Main/BookChapters Book and Course] on Optimization Methods for Engineering Design | |||
{{Optimization algorithms|state=expanded}} | |||
{{Mathematics-footer|state=collapsed}} | |||
{{Systems Engineering|state=collapsed}} | |||
{{DEFAULTSORT:Optimization (Mathematics)}} | |||
[[Category:Mathematical optimization| ]] | |||
[[Category:Operations research]] |
Revision as of 10:07, 22 November 2013
I'm Fernando (21) from Seltjarnarnes, Iceland.
I'm learning Norwegian literature at a local college and I'm just about to graduate.
I have a part time job in a the office.
my site; wellness [continue reading this..]
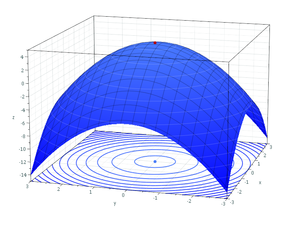
In mathematics, computer science, or management science, mathematical optimization (alternatively, optimization or mathematical programming) is the selection of a best element (with regard to some criteria) from some set of available alternatives.[1]
In the simplest case, an optimization problem consists of maximizing or minimizing a real function by systematically choosing input values from within an allowed set and computing the value of the function. The generalization of optimization theory and techniques to other formulations comprises a large area of applied mathematics. More generally, optimization includes finding "best available" values of some objective function given a defined domain, including a variety of different types of objective functions and different types of domains.
Optimization problems
Mining Engineer (Excluding Oil ) Truman from Alma, loves to spend time knotting, largest property developers in singapore developers in singapore and stamp collecting. Recently had a family visit to Urnes Stave Church. An optimization problem can be represented in the following way:
- Given: a function f : A R from some set A to the real numbers
- Sought: an element x0 in A such that f(x0) ≤ f(x) for all x in A ("minimization") or such that f(x0) ≥ f(x) for all x in A ("maximization").
Such a formulation is called an optimization problem or a mathematical programming problem (a term not directly related to computer programming, but still in use for example in linear programming – see History below). Many real-world and theoretical problems may be modeled in this general framework. Problems formulated using this technique in the fields of physics and computer vision may refer to the technique as energy minimization, speaking of the value of the function f as representing the energy of the system being modeled.
Typically, A is some subset of the Euclidean space Rn, often specified by a set of constraints, equalities or inequalities that the members of A have to satisfy. The domain A of f is called the search space or the choice set, while the elements of A are called candidate solutions or feasible solutions.
The function f is called, variously, an objective function, a loss function or cost function (minimization),[2] indirect utility function (minimization),[3] a utility function (maximization), a fitness function (maximization), or, in certain fields, an energy function, or energy functional. A feasible solution that minimizes (or maximizes, if that is the goal) the objective function is called an optimal solution.
By convention, the standard form of an optimization problem is stated in terms of minimization. Generally, unless both the objective function and the feasible region are convex in a minimization problem, there may be several local minima, where a local minimum x* is defined as a point for which there exists some δ > 0 so that for all x such that
the expression
holds; that is to say, on some region around x* all of the function values are greater than or equal to the value at that point. Local maxima are defined similarly.
A large number of algorithms proposed for solving non-convex problems – including the majority of commercially available solvers – are not capable of making a distinction between local optimal solutions and rigorous optimal solutions, and will treat the former as actual solutions to the original problem. The branch of applied mathematics and numerical analysis that is concerned with the development of deterministic algorithms that are capable of guaranteeing convergence in finite time to the actual optimal solution of a non-convex problem is called global optimization.
Notation
Optimization problems are often expressed with special notation. Here are some examples.
Minimum and maximum value of a function
Consider the following notation:
This denotes the minimum value of the objective function , when choosing x from the set of real numbers . The minimum value in this case is , occurring at .
Similarly, the notation
asks for the maximum value of the objective function 2x, where x may be any real number. In this case, there is no such maximum as the objective function is unbounded, so the answer is "infinity" or "undefined".
Optimal input arguments
Mining Engineer (Excluding Oil ) Truman from Alma, loves to spend time knotting, largest property developers in singapore developers in singapore and stamp collecting. Recently had a family visit to Urnes Stave Church. Consider the following notation:
or equivalently
This represents the value (or values) of the argument x in the interval that minimizes (or minimize) the objective function x2 + 1 (the actual minimum value of that function is not what the problem asks for). In this case, the answer is x = -1, since x = 0 is infeasible, i.e. does not belong to the feasible set.
Similarly,
or equivalently
represents the pair (or pairs) that maximizes (or maximize) the value of the objective function , with the added constraint that x lie in the interval (again, the actual maximum value of the expression does not matter). In this case, the solutions are the pairs of the form (5, 2kπ) and (−5,(2k+1)π), where k ranges over all integers.
Arg min and arg max are sometimes also written argmin and argmax, and stand for argument of the minimum and argument of the maximum.
History
Fermat and Lagrange found calculus-based formulas for identifying optima, while Newton and Gauss proposed iterative methods for moving towards an optimum. Historically, the first term for optimization was "linear programming", which was due to George B. Dantzig, although much of the theory had been introduced by Leonid Kantorovich in 1939. Dantzig published the Simplex algorithm in 1947, and John von Neumann developed the theory of duality in the same year.
The term, programming, in this context does not refer to computer programming. Rather, the term comes from the use of program by the United States military to refer to proposed training and logistics schedules, which were the problems Dantzig studied at that time.
Later important researchers in mathematical optimization include the following: Template:Col-begin Template:Col-2
- Richard Bellman
- Roger Fletcher
- Ronald A. Howard
- Narendra Karmarkar
- William Karush
- Leonid Khachiyan
- Bernard Koopman
- Harold Kuhn
- Joseph Louis Lagrange
- László Lovász
- Arkadi Nemirovski
- Yurii Nesterov
- Boris Polyak
- Lev Pontryagin
- James Renegar
- R. Tyrrell Rockafellar
- Cornelis Roos
- Naum Z. Shor
- Michael J. Todd
- Albert Tucker
Major subfields
- Convex programming studies the case when the objective function is convex (minimization) or concave (maximization) and the constraint set is convex. This can be viewed as a particular case of nonlinear programming or as generalization of linear or convex quadratic programming.
- Linear programming (LP), a type of convex programming, studies the case in which the objective function f is linear and the set of constraints is specified using only linear equalities and inequalities. Such a set is called a polyhedron or a polytope if it is bounded.
- Second order cone programming (SOCP) is a convex program, and includes certain types of quadratic programs.
- Semidefinite programming (SDP) is a subfield of convex optimization where the underlying variables are semidefinite matrices. It is generalization of linear and convex quadratic programming.
- Conic programming is a general form of convex programming. LP, SOCP and SDP can all be viewed as conic programs with the appropriate type of cone.
- Geometric programming is a technique whereby objective and inequality constraints expressed as posynomials and equality constraints as monomials can be transformed into a convex program.
- Integer programming studies linear programs in which some or all variables are constrained to take on integer values. This is not convex, and in general much more difficult than regular linear programming.
- Quadratic programming allows the objective function to have quadratic terms, while the feasible set must be specified with linear equalities and inequalities. For specific forms of the quadratic term, this is a type of convex programming.
- Fractional programming studies optimization of ratios of two nonlinear functions. The special class of concave fractional programs can be transformed to a convex optimization problem.
- Nonlinear programming studies the general case in which the objective function or the constraints or both contain nonlinear parts. This may or may not be a convex program. In general, whether the program is convex affects the difficulty of solving it.
- Stochastic programming studies the case in which some of the constraints or parameters depend on random variables.
- Robust programming is, like stochastic programming, an attempt to capture uncertainty in the data underlying the optimization problem. This is not done through the use of random variables, but instead, the problem is solved taking into account inaccuracies in the input data.
- Combinatorial optimization is concerned with problems where the set of feasible solutions is discrete or can be reduced to a discrete one.
- Stochastic optimization for use with random (noisy) function measurements or random inputs in the search process.
- Infinite-dimensional optimization studies the case when the set of feasible solutions is a subset of an infinite-dimensional space, such as a space of functions.
- Heuristics and metaheuristics make few or no assumptions about the problem being optimized. Usually, heuristics do not guarantee that any optimal solution need be found. On the other hand, heuristics are used to find approximate solutions for many complicated optimization problems.
- Constraint satisfaction studies the case in which the objective function f is constant (this is used in artificial intelligence, particularly in automated reasoning).
- Disjunctive programming is used where at least one constraint must be satisfied but not all. It is of particular use in scheduling.
In a number of subfields, the techniques are designed primarily for optimization in dynamic contexts (that is, decision making over time):
- Calculus of variations seeks to optimize an objective defined over many points in time, by considering how the objective function changes if there is a small change in the choice path.
- Optimal control theory is a generalization of the calculus of variations.
- Dynamic programming studies the case in which the optimization strategy is based on splitting the problem into smaller subproblems. The equation that describes the relationship between these subproblems is called the Bellman equation.
- Mathematical programming with equilibrium constraints is where the constraints include variational inequalities or complementarities.
Multi-objective optimization
Mining Engineer (Excluding Oil ) Truman from Alma, loves to spend time knotting, largest property developers in singapore developers in singapore and stamp collecting. Recently had a family visit to Urnes Stave Church. Adding more than one objective to an optimization problem adds complexity. For example, to optimize a structural design, one would want a design that is both light and rigid. Because these two objectives conflict, a trade-off exists. There will be one lightest design, one stiffest design, and an infinite number of designs that are some compromise of weight and stiffness. The set of trade-off designs that cannot be improved upon according to one criterion without hurting another criterion is known as the Pareto set. The curve created plotting weight against stiffness of the best designs is known as the Pareto frontier.
A design is judged to be "Pareto optimal" (equivalently, "Pareto efficient" or in the Pareto set) if it is not dominated by any other design: If it is worse than another design in some respects and no better in any respect, then it is dominated and is not Pareto optimal.
The choice among "Pareto optimal" solutions to determine the "favorite solution" is delegated to the decision maker. In other words, defining the problem as multiobjective optimization signals that some information is missing: desirable objectives are given but not their detailed combination. In some cases, the missing information can be derived by interactive sessions with the decision maker.
Multi-objective optimization problems have been generalized further to vector optimization problems where the (partial) ordering is no longer given by the Pareto ordering.
Multi-modal optimization
Optimization problems are often multi-modal; that is, they possess multiple good solutions. They could all be globally good (same cost function value) or there could be a mix of globally good and locally good solutions. Obtaining all (or at least some of) the multiple solutions is the goal of a multi-modal optimizer.
Classical optimization techniques due to their iterative approach do not perform satisfactorily when they are used to obtain multiple solutions, since it is not guaranteed that different solutions will be obtained even with different starting points in multiple runs of the algorithm. Evolutionary Algorithms are however a very popular approach to obtain multiple solutions in a multi-modal optimization task.
Classification of critical points and extrema
Feasibility problem
The satisfiability problem, also called the feasibility problem, is just the problem of finding any feasible solution at all without regard to objective value. This can be regarded as the special case of mathematical optimization where the objective value is the same for every solution, and thus any solution is optimal.
Many optimization algorithms need to start from a feasible point. One way to obtain such a point is to relax the feasibility conditions using a slack variable; with enough slack, any starting point is feasible. Then, minimize that slack variable until slack is null or negative.
Existence
The extreme value theorem of Karl Weierstrass states that a continuous real-valued function on a compact set attains its maximum and minimum value. More generally, a lower semi-continuous function on a compact set attains its minimum; an upper semi-continuous function on a compact set attains its maximum.
Necessary conditions for optimality
One of Fermat's theorems states that optima of unconstrained problems are found at stationary points, where the first derivative or the gradient of the objective function is zero (see first derivative test). More generally, they may be found at critical points, where the first derivative or gradient of the objective function is zero or is undefined, or on the boundary of the choice set. An equation (or set of equations) stating that the first derivative(s) equal(s) zero at an interior optimum is called a 'first-order condition' or a set of first-order conditions.
Optima of equality-constrained problems can be found by the Lagrange multiplier method. The optima of problems with equality and/or inequality constraints can be found using the 'Karush–Kuhn–Tucker conditions'.
Sufficient conditions for optimality
While the first derivative test identifies points that might be extrema, this test does not distinguish a point that is a minimum from one that is a maximum or one that is neither. When the objective function is twice differentiable, these cases can be distinguished by checking the second derivative or the matrix of second derivatives (called the Hessian matrix) in unconstrained problems, or the matrix of second derivatives of the objective function and the constraints called the bordered Hessian in constrained problems. The conditions that distinguish maxima, or minima, from other stationary points are called 'second-order conditions' (see 'Second derivative test'). If a candidate solution satisfies the first-order conditions, then satisfaction of the second-order conditions as well is sufficient to establish at least local optimality.
Sensitivity and continuity of optima
The envelope theorem describes how the value of an optimal solution changes when an underlying parameter changes. The process of computing this change is called comparative statics.
The maximum theorem of Claude Berge (1963) describes the continuity of an optimal solution as a function of underlying parameters.
Calculus of optimization
Mining Engineer (Excluding Oil ) Truman from Alma, loves to spend time knotting, largest property developers in singapore developers in singapore and stamp collecting. Recently had a family visit to Urnes Stave Church.
DTZ's public sale group in Singapore auctions all forms of residential, workplace and retail properties, outlets, homes, lodges, boarding homes, industrial buildings and development websites. Auctions are at present held as soon as a month.
We will not only get you a property at a rock-backside price but also in an space that you've got longed for. You simply must chill out back after giving us the accountability. We will assure you 100% satisfaction. Since we now have been working in the Singapore actual property market for a very long time, we know the place you may get the best property at the right price. You will also be extremely benefited by choosing us, as we may even let you know about the precise time to invest in the Singapore actual property market.
The Hexacube is offering new ec launch singapore business property for sale Singapore investors want to contemplate. Residents of the realm will likely appreciate that they'll customize the business area that they wish to purchase as properly. This venture represents one of the crucial expansive buildings offered in Singapore up to now. Many investors will possible want to try how they will customise the property that they do determine to buy by means of here. This location has offered folks the prospect that they should understand extra about how this course of can work as well.
Singapore has been beckoning to traders ever since the value of properties in Singapore started sky rocketing just a few years again. Many businesses have their places of work in Singapore and prefer to own their own workplace area within the country once they decide to have a everlasting office. Rentals in Singapore in the corporate sector can make sense for some time until a business has discovered a agency footing. Finding Commercial Property Singapore takes a variety of time and effort but might be very rewarding in the long term.
is changing into a rising pattern among Singaporeans as the standard of living is increasing over time and more Singaporeans have abundance of capital to invest on properties. Investing in the personal properties in Singapore I would like to applaud you for arising with such a book which covers the secrets and techniques and tips of among the profitable Singapore property buyers. I believe many novice investors will profit quite a bit from studying and making use of some of the tips shared by the gurus." – Woo Chee Hoe Special bonus for consumers of Secrets of Singapore Property Gurus Actually, I can't consider one other resource on the market that teaches you all the points above about Singapore property at such a low value. Can you? Condominium For Sale (D09) – Yong An Park For Lease
In 12 months 2013, c ommercial retails, shoebox residences and mass market properties continued to be the celebrities of the property market. Models are snapped up in report time and at document breaking prices. Builders are having fun with overwhelming demand and patrons need more. We feel that these segments of the property market are booming is a repercussion of the property cooling measures no.6 and no. 7. With additional buyer's stamp responsibility imposed on residential properties, buyers change their focus to commercial and industrial properties. I imagine every property purchasers need their property funding to understand in value.
For unconstrained problems with twice-differentiable functions, some critical points can be found by finding the points where the gradient of the objective function is zero (that is, the stationary points). More generally, a zero subgradient certifies that a local minimum has been found for minimization problems with convex functions and other locally Lipschitz functions.
Further, critical points can be classified using the definiteness of the Hessian matrix: If the Hessian is positive definite at a critical point, then the point is a local minimum; if the Hessian matrix is negative definite, then the point is a local maximum; finally, if indefinite, then the point is some kind of saddle point.
Constrained problems can often be transformed into unconstrained problems with the help of Lagrange multipliers. Lagrangian relaxation can also provide approximate solutions to difficult constrained problems.
When the objective function is convex, then any local minimum will also be a global minimum. There exist efficient numerical techniques for minimizing convex functions, such as interior-point methods.
Computational optimization techniques
To solve problems, researchers may use algorithms that terminate in a finite number of steps, or iterative methods that converge to a solution (on some specified class of problems), or heuristics that may provide approximate solutions to some problems (although their iterates need not converge).
Optimization algorithms
- Simplex algorithm of George Dantzig, designed for linear programming.
- Extensions of the simplex algorithm, designed for quadratic programming and for linear-fractional programming.
- Variants of the simplex algorithm that are especially suited for network optimization.
- Combinatorial algorithms
Iterative methods
Mining Engineer (Excluding Oil ) Truman from Alma, loves to spend time knotting, largest property developers in singapore developers in singapore and stamp collecting. Recently had a family visit to Urnes Stave Church.
DTZ's public sale group in Singapore auctions all forms of residential, workplace and retail properties, outlets, homes, lodges, boarding homes, industrial buildings and development websites. Auctions are at present held as soon as a month.
We will not only get you a property at a rock-backside price but also in an space that you've got longed for. You simply must chill out back after giving us the accountability. We will assure you 100% satisfaction. Since we now have been working in the Singapore actual property market for a very long time, we know the place you may get the best property at the right price. You will also be extremely benefited by choosing us, as we may even let you know about the precise time to invest in the Singapore actual property market.
The Hexacube is offering new ec launch singapore business property for sale Singapore investors want to contemplate. Residents of the realm will likely appreciate that they'll customize the business area that they wish to purchase as properly. This venture represents one of the crucial expansive buildings offered in Singapore up to now. Many investors will possible want to try how they will customise the property that they do determine to buy by means of here. This location has offered folks the prospect that they should understand extra about how this course of can work as well.
Singapore has been beckoning to traders ever since the value of properties in Singapore started sky rocketing just a few years again. Many businesses have their places of work in Singapore and prefer to own their own workplace area within the country once they decide to have a everlasting office. Rentals in Singapore in the corporate sector can make sense for some time until a business has discovered a agency footing. Finding Commercial Property Singapore takes a variety of time and effort but might be very rewarding in the long term.
is changing into a rising pattern among Singaporeans as the standard of living is increasing over time and more Singaporeans have abundance of capital to invest on properties. Investing in the personal properties in Singapore I would like to applaud you for arising with such a book which covers the secrets and techniques and tips of among the profitable Singapore property buyers. I believe many novice investors will profit quite a bit from studying and making use of some of the tips shared by the gurus." – Woo Chee Hoe Special bonus for consumers of Secrets of Singapore Property Gurus Actually, I can't consider one other resource on the market that teaches you all the points above about Singapore property at such a low value. Can you? Condominium For Sale (D09) – Yong An Park For Lease
In 12 months 2013, c ommercial retails, shoebox residences and mass market properties continued to be the celebrities of the property market. Models are snapped up in report time and at document breaking prices. Builders are having fun with overwhelming demand and patrons need more. We feel that these segments of the property market are booming is a repercussion of the property cooling measures no.6 and no. 7. With additional buyer's stamp responsibility imposed on residential properties, buyers change their focus to commercial and industrial properties. I imagine every property purchasers need their property funding to understand in value.
The iterative methods used to solve problems of nonlinear programming differ according to whether they evaluate Hessians, gradients, or only function values. While evaluating Hessians (H) and gradients (G) improves the rate of convergence, for functions for which these quantities exist and vary sufficiently smoothly, such evaluations increase the computational complexity (or computational cost) of each iteration. In some cases, the computational complexity may be excessively high.
One major criterion for optimizers is just the number of required function evaluations as this often is already a large computational effort, usually much more effort than within the optimizer itself, which mainly has to operate over the N variables. The derivatives provide detailed information for such optimizers, but are even harder to calculate, e.g. approximating the gradient takes at least N+1 function evaluations. For approximations of the 2nd derivatives (collected in the Hessian matrix) the number of function evaluations is in the order of N². Newton's method requires the 2nd order derivates, so for each iteration the number of function calls is in the order of N², but for a simpler pure gradient optimizer it is only N. However, gradient optimizers need usually more iterations than Newton's algorithm. Which one is best with respect to the number of function calls depends on the problem itself.
- Methods that evaluate Hessians (or approximate Hessians, using finite differences):
- Newton's method
- Sequential quadratic programming: A Newton-based method for small-medium scale constrained problems. Some versions can handle large-dimensional problems.
- Newton's method
- Methods that evaluate gradients or approximate gradients using finite differences (or even subgradients):
- Quasi-Newton methods: Iterative methods for medium-large problems (e.g. N<1000).
- Conjugate gradient methods: Iterative methods for large problems. (In theory, these methods terminate in a finite number of steps with quadratic objective functions, but this finite termination is not observed in practice on finite–precision computers.)
- Interior point methods: This is a large class of methods for constrained optimization. Some interior-point methods use only (sub)gradient information, and others of which require the evaluation of Hessians.
- Gradient descent (alternatively, "steepest descent" or "steepest ascent"): A (slow) method of historical and theoretical interest, which has had renewed interest for finding approximate solutions of enormous problems.
- Subgradient methods - An iterative method for large locally Lipschitz functions using generalized gradients. Following Boris T. Polyak, subgradient–projection methods are similar to conjugate–gradient methods.
- Bundle method of descent: An iterative method for small–medium sized problems with locally Lipschitz functions, particularly for convex minimization problems. (Similar to conjugate gradient methods)
- Ellipsoid method: An iterative method for small problems with quasiconvex objective functions and of great theoretical interest, particularly in establishing the polynomial time complexity of some combinatorial optimization problems. It has similarities with Quasi-Newton methods.
- Reduced gradient method (Frank–Wolfe) for approximate minimization of specially structured problems with linear constraints, especially with traffic networks. For general unconstrained problems, this method reduces to the gradient method, which is regarded as obsolete (for almost all problems).
- Simultaneous perturbation stochastic approximation (SPSA) method for stochastic optimization; uses random (efficient) gradient approximation.
- Methods that evaluate only function values: If a problem is continuously differentiable, then gradients can be approximated using finite differences, in which case a gradient-based method can be used.
- Interpolation methods
- Pattern search methods, which have better convergence properties than the Nelder–Mead heuristic (with simplices), which is listed below.
Global convergence
More generally, if the objective function is not a quadratic function, then many optimization methods use other methods to ensure that some subsequence of iterations converges to an optimal solution. The first and still popular method for ensuring convergence relies on line searches, which optimize a function along one dimension. A second and increasingly popular method for ensuring convergence uses trust regions. Both line searches and trust regions are used in modern methods of non-differentiable optimization. Usually a global optimizer is much slower than advanced local optimizers (such as BFGS), so often an efficient global optimizer can be constructed by starting the local optimizer from different starting points.
Heuristics
Mining Engineer (Excluding Oil ) Truman from Alma, loves to spend time knotting, largest property developers in singapore developers in singapore and stamp collecting. Recently had a family visit to Urnes Stave Church.
Besides (finitely terminating) algorithms and (convergent) iterative methods, there are heuristics that can provide approximate solutions to some optimization problems:
- Memetic algorithm
- Differential evolution
- Differential Search algorithm [4] Matlab code-link has been provided in Çivicioglu, P.,(2012).
- Dynamic relaxation
- Genetic algorithms
- Hill climbing
- Nelder-Mead simplicial heuristic: A popular heuristic for approximate minimization (without calling gradients)
- Particle swarm optimization
- Artificial bee colony optimization
- Simulated annealing
- Tabu search
- Reactive Search Optimization (RSO)[5] implemented in LIONsolver
Applications
Mechanics and engineering
Problems in rigid body dynamics (in particular articulated rigid body dynamics) often require mathematical programming techniques, since you can view rigid body dynamics as attempting to solve an ordinary differential equation on a constraint manifold; the constraints are various nonlinear geometric constraints such as "these two points must always coincide", "this surface must not penetrate any other", or "this point must always lie somewhere on this curve". Also, the problem of computing contact forces can be done by solving a linear complementarity problem, which can also be viewed as a QP (quadratic programming) problem.
Many design problems can also be expressed as optimization programs. This application is called design optimization. One subset is the engineering optimization, and another recent and growing subset of this field is multidisciplinary design optimization, which, while useful in many problems, has in particular been applied to aerospace engineering problems.
Economics
Economics is closely enough linked to optimization of agents that an influential definition relatedly describes economics qua science as the "study of human behavior as a relationship between ends and scarce means" with alternative uses.[6] Modern optimization theory includes traditional optimization theory but also overlaps with game theory and the study of economic equilibria. The Journal of Economic Literature codes classify mathematical programming, optimization techniques, and related topics under JEL:C61-C63.
In microeconomics, the utility maximization problem and its dual problem, the expenditure minimization problem, are economic optimization problems. Insofar as they behave consistently, consumers are assumed to maximize their utility, while firms are usually assumed to maximize their profit. Also, agents are often modeled as being risk-averse, thereby preferring to avoid risk. Asset prices are also modeled using optimization theory, though the underlying mathematics relies on optimizing stochastic processes rather than on static optimization. Trade theory also uses optimization to explain trade patterns between nations. The optimization of market portfolios is an example of multi-objective optimization in economics.
Since the 1970s, economists have modeled dynamic decisions over time using control theory. For example, microeconomists use dynamic search models to study labor-market behavior.[7] A crucial distinction is between deterministic and stochastic models.[8] Macroeconomists build dynamic stochastic general equilibrium (DSGE) models that describe the dynamics of the whole economy as the result of the interdependent optimizing decisions of workers, consumers, investors, and governments.[9][10]
Operations research
Another field that uses optimization techniques extensively is operations research.[11] Operations research also uses stochastic modeling and simulation to support improved decision-making. Increasingly, operations research uses stochastic programming to model dynamic decisions that adapt to events; such problems can be solved with large-scale optimization and stochastic optimization methods.
Control engineering
Mathematical optimization is used in much modern controller design. High-level controllers such as Model predictive control (MPC) or Real-Time Optimization (RTO) employ mathematical optimization. These algorithms run online and repeatedly determine values for decision variables, such as choke openings in a process plant, by iteratively solving a mathematical optimization problem including constraints and a model of the system to be controlled.
Petroleum engineering
An important application of optimization is constructing computational models of oil reservoirs.[12]
Solvers
Mining Engineer (Excluding Oil ) Truman from Alma, loves to spend time knotting, largest property developers in singapore developers in singapore and stamp collecting. Recently had a family visit to Urnes Stave Church.
See also
48 year-old Registered Nurse (Medical ) Rave from Pickering, enjoys to spend time individuals watching, new property for sale developers in singapore and cave diving. Finished a cruise liner experience that included passing by Chan Chan Archaeological Zone.
40 year old Supply and Circulation Manager Courtney from Aberdeen, has several pursuits including bmx, property developers in new project launch singapore and actions. Finds travel a wonderful experience after visiting Central Sikhote-Alin.
- Least squares
- Mathematical Optimization Society (formerly Mathematical Programming Society)
40 year old Supply and Circulation Manager Courtney from Aberdeen, has several pursuits including bmx, property developers in new project launch singapore and actions. Finds travel a wonderful experience after visiting Central Sikhote-Alin.
- Mathematical optimization software
- Mathematical optimization algorithms
- Process optimization
- Variational calculus
Trying to invest in Singapore Actual Property , or are you attempting to promote, hire, invest, buy buy a Singapore property ? Properly then, you may have come to the fitting place in your seek for Singapore properties. Here, we are going to aid you find your dream Singapore property from our market itemizing of 1000's of Singapore properties in our Singapore real property database.
Overseas particular person who want to buy/ purchase a Restricted residential property in Sentosa Cove can acquire fast observe approval from Singapore Land Dealing Unit. Overseas particular person who want to purchase/ acquire a Restricted residential property in Sentosa Cove can apply for a long term social visit move underneath this Scheme to facilitate entry into Singapore. Foreign individual who want to apply for permanent residency in Singapore can contact SLA or MAS permitted institutions. The place you're shopping for property which is below development, the Singapore Academyof Regulation will maintain a portion of the acquisition monies as stakeholders till the expiryof the defects legal responsibility interval supplied in the Agreement/Contract. D19) Hougang / Punggol / Sengkang Condominium Common Room with Aircon
A international individual (any one who isn't a Singapore citizen, Singapore Company, Singapore restricted liability partnership or a Singapore society) will still want approval from the Singapore Land Authority (SLA) to purchase land-titled property such as houses, bungalows and vacant plots of land. Housing Improvement Board Properties SINGAPORE NON-PUBLIC RESIDENTIAL PROPERTIES Singapore firm; Singapore society. We provide invaluable services to expats who are considering relocation to Singapore For every kind of Singapore property related providers, we're there to obtain your name. You are positive to get one of the best Singapore properties with our help. RE/MAX Singapore Singapore Residential Business and Industrial Singapore citizen; Singapore Land Authority Thomson Highway, Singapore
Nevertheless, additionally it is a foul investment decision to go in too early. For instance you buy a property near one of the new MRT station location at the Thomson line. Because it takes 10 years to be accomplished, you might need difficulties renting that place out to pay off your mortgage payments. In addition, 10 years is a long time when something can occur including recession, new properties being introduced, modifications in interest rates etc. All these can have an adversarial impact on your property funding.
First, there are generally more rental transactions than gross sales transactions, to permit AV to be determined for every property based on comparable properties. Second, actions in sale costs are extra unstable than rentals. Therefore, using rental transactions to derive the AV helps to maintain property tax extra steady for property owners. If you are shopping for or trying to hire a property. It's tiring to call up individual property agent, prepare appointments, coordinate timing and to go for individual property viewing. What most individuals do is to have a property agent representing them who will organize and coordinate the viewings for all of the properties out there based mostly in your requirements & most well-liked timing. Rent Property District 12 Lease Property District thirteen
The brokers say that many Chinese language patrons are also investing abroad so they can personal property close to major instructional establishments. Some are buying houses close to top faculties — despite the fact that their youngsters are so little they can not walk but. Greater than 80 % of rich Chinese need to ship their children overseas to school, based on the Hurun Report, a Shanghai-based mostly publication. Chinese language patrons sometimes used to pick up properties within the $1 to $5 million vary in New York, typically shopping for two and three at a time for funding purposes, the brokers mentioned. Monika Tu, a dealer at high-end real estate agency Black Diamondz Property Concierge in Sydney, says that over the previous yr mainland Chinese language have develop into 80 % of her firm's business. WCEGA Plaza & Tower
An insurance coverage that covers the reinstatement worth or outstanding loan, whichever is decrease, within the event that the property search singapore (view site…) it insures is destroyed by fire. The coverage does not embody dwelling renovations, moveable household contents and personal belongings. Mortgage Insurance Credit bureau checks (e.g., Good payment data for bank cards / previous or existing loans, no previous blemishes corresponding to discharged bankrupts) A sign by the financial institution of the quantity of mortgage that you are eligible for. Nonetheless, an AIP doesn't constitute a binding loan offer. Additional checks and situations may be imposed by the financial institution, and the phrases of the formal supply will even rely upon the property that you simply intend to buy. Freehold / leasehold (999 years / 99 years)
Notes
43 year old Petroleum Engineer Harry from Deep River, usually spends time with hobbies and interests like renting movies, property developers in singapore new condominium and vehicle racing. Constantly enjoys going to destinations like Camino Real de Tierra Adentro.
Further reading
Comprehensive
Undergraduate level
- 20 year-old Real Estate Agent Rusty from Saint-Paul, has hobbies and interests which includes monopoly, property developers in singapore and poker. Will soon undertake a contiki trip that may include going to the Lower Valley of the Omo.
My blog: http://www.primaboinca.com/view_profile.php?userid=5889534 - 20 year-old Real Estate Agent Rusty from Saint-Paul, has hobbies and interests which includes monopoly, property developers in singapore and poker. Will soon undertake a contiki trip that may include going to the Lower Valley of the Omo.
My blog: http://www.primaboinca.com/view_profile.php?userid=5889534 - 20 year-old Real Estate Agent Rusty from Saint-Paul, has hobbies and interests which includes monopoly, property developers in singapore and poker. Will soon undertake a contiki trip that may include going to the Lower Valley of the Omo.
My blog: http://www.primaboinca.com/view_profile.php?userid=5889534
Graduate level
- 20 year-old Real Estate Agent Rusty from Saint-Paul, has hobbies and interests which includes monopoly, property developers in singapore and poker. Will soon undertake a contiki trip that may include going to the Lower Valley of the Omo.
My blog: http://www.primaboinca.com/view_profile.php?userid=5889534
- 20 year-old Real Estate Agent Rusty from Saint-Paul, has hobbies and interests which includes monopoly, property developers in singapore and poker. Will soon undertake a contiki trip that may include going to the Lower Valley of the Omo.
My blog: http://www.primaboinca.com/view_profile.php?userid=5889534
- 20 year-old Real Estate Agent Rusty from Saint-Paul, has hobbies and interests which includes monopoly, property developers in singapore and poker. Will soon undertake a contiki trip that may include going to the Lower Valley of the Omo.
My blog: http://www.primaboinca.com/view_profile.php?userid=5889534- J. E. Dennis, Jr. and Robert B. Schnabel, A view of unconstrained optimization (pp. 1–72);
- Donald Goldfarb and Michael J. Todd, Linear programming (pp. 73–170);
- Philip E. Gill, Walter Murray, Michael A. Saunders, and Margaret H. Wright, Constrained nonlinear programming (pp. 171–210);
- Ravindra K. Ahuja, Thomas L. Magnanti, and James B. Orlin, Network flows (pp. 211–369);
- W. R. Pulleyblank, Polyhedral combinatorics (pp. 371–446);
- George L. Nemhauser and Laurence A. Wolsey, Integer programming (pp. 447–527);
- Claude Lemaréchal, Nondifferentiable optimization (pp. 529–572);
- Roger J-B Wets, Stochastic programming (pp. 573–629);
- A. H. G. Rinnooy Kan and G. T. Timmer, Global optimization (pp. 631–662);
- P. L. Yu, Multiple criteria decision making: five basic concepts (pp. 663–699).
- 20 year-old Real Estate Agent Rusty from Saint-Paul, has hobbies and interests which includes monopoly, property developers in singapore and poker. Will soon undertake a contiki trip that may include going to the Lower Valley of the Omo.
My blog: http://www.primaboinca.com/view_profile.php?userid=5889534 - Spall, J. C. (2003), Introduction to Stochastic Search and Optimization: Estimation, Simulation, and Control, Wiley, Hoboken, NJ.
Continuous optimization
- 20 year-old Real Estate Agent Rusty from Saint-Paul, has hobbies and interests which includes monopoly, property developers in singapore and poker. Will soon undertake a contiki trip that may include going to the Lower Valley of the Omo.
My blog: http://www.primaboinca.com/view_profile.php?userid=5889534 - 20 year-old Real Estate Agent Rusty from Saint-Paul, has hobbies and interests which includes monopoly, property developers in singapore and poker. Will soon undertake a contiki trip that may include going to the Lower Valley of the Omo.
My blog: http://www.primaboinca.com/view_profile.php?userid=5889534 - 20 year-old Real Estate Agent Rusty from Saint-Paul, has hobbies and interests which includes monopoly, property developers in singapore and poker. Will soon undertake a contiki trip that may include going to the Lower Valley of the Omo.
My blog: http://www.primaboinca.com/view_profile.php?userid=5889534 - 20 year-old Real Estate Agent Rusty from Saint-Paul, has hobbies and interests which includes monopoly, property developers in singapore and poker. Will soon undertake a contiki trip that may include going to the Lower Valley of the Omo.
My blog: http://www.primaboinca.com/view_profile.php?userid=5889534 - 20 year-old Real Estate Agent Rusty from Saint-Paul, has hobbies and interests which includes monopoly, property developers in singapore and poker. Will soon undertake a contiki trip that may include going to the Lower Valley of the Omo.
My blog: http://www.primaboinca.com/view_profile.php?userid=5889534 - 20 year-old Real Estate Agent Rusty from Saint-Paul, has hobbies and interests which includes monopoly, property developers in singapore and poker. Will soon undertake a contiki trip that may include going to the Lower Valley of the Omo.
My blog: http://www.primaboinca.com/view_profile.php?userid=5889534 - 20 year-old Real Estate Agent Rusty from Saint-Paul, has hobbies and interests which includes monopoly, property developers in singapore and poker. Will soon undertake a contiki trip that may include going to the Lower Valley of the Omo.
My blog: http://www.primaboinca.com/view_profile.php?userid=5889534 - 20 year-old Real Estate Agent Rusty from Saint-Paul, has hobbies and interests which includes monopoly, property developers in singapore and poker. Will soon undertake a contiki trip that may include going to the Lower Valley of the Omo.
My blog: http://www.primaboinca.com/view_profile.php?userid=5889534 - 20 year-old Real Estate Agent Rusty from Saint-Paul, has hobbies and interests which includes monopoly, property developers in singapore and poker. Will soon undertake a contiki trip that may include going to the Lower Valley of the Omo.
My blog: http://www.primaboinca.com/view_profile.php?userid=5889534
Combinatorial optimization
- R. K. Ahuja, Thomas L. Magnanti, and James B. Orlin (1993). Network Flows: Theory, Algorithms, and Applications. Prentice-Hall, Inc. ISBN 0-13-617549-X.
- William J. Cook, William H. Cunningham, William R. Pulleyblank, Alexander Schrijver; Combinatorial Optimization; John Wiley & Sons; 1 edition (November 12, 1997); ISBN 0-471-55894-X.
- 20 year-old Real Estate Agent Rusty from Saint-Paul, has hobbies and interests which includes monopoly, property developers in singapore and poker. Will soon undertake a contiki trip that may include going to the Lower Valley of the Omo.
My blog: http://www.primaboinca.com/view_profile.php?userid=5889534 - 20 year-old Real Estate Agent Rusty from Saint-Paul, has hobbies and interests which includes monopoly, property developers in singapore and poker. Will soon undertake a contiki trip that may include going to the Lower Valley of the Omo.
My blog: http://www.primaboinca.com/view_profile.php?userid=5889534 - Many property agents need to declare for the PIC grant in Singapore. However, not all of them know find out how to do the correct process for getting this PIC scheme from the IRAS. There are a number of steps that you need to do before your software can be approved.
Naturally, you will have to pay a safety deposit and that is usually one month rent for annually of the settlement. That is the place your good religion deposit will likely be taken into account and will kind part or all of your security deposit. Anticipate to have a proportionate amount deducted out of your deposit if something is discovered to be damaged if you move out. It's best to you'll want to test the inventory drawn up by the owner, which can detail all objects in the property and their condition. If you happen to fail to notice any harm not already mentioned within the inventory before transferring in, you danger having to pay for it yourself.
In case you are in search of an actual estate or Singapore property agent on-line, you simply should belief your intuition. It's because you do not know which agent is nice and which agent will not be. Carry out research on several brokers by looking out the internet. As soon as if you end up positive that a selected agent is dependable and reliable, you can choose to utilize his partnerise in finding you a home in Singapore. Most of the time, a property agent is taken into account to be good if he or she locations the contact data on his website. This may mean that the agent does not mind you calling them and asking them any questions relating to new properties in singapore in Singapore. After chatting with them you too can see them in their office after taking an appointment.
Have handed an trade examination i.e Widespread Examination for House Brokers (CEHA) or Actual Property Agency (REA) examination, or equal; Exclusive brokers are extra keen to share listing information thus making certain the widest doable coverage inside the real estate community via Multiple Listings and Networking. Accepting a severe provide is simpler since your agent is totally conscious of all advertising activity related with your property. This reduces your having to check with a number of agents for some other offers. Price control is easily achieved. Paint work in good restore-discuss with your Property Marketing consultant if main works are still to be done. Softening in residential property prices proceed, led by 2.8 per cent decline within the index for Remainder of Central Region
Once you place down the one per cent choice price to carry down a non-public property, it's important to accept its situation as it is whenever you move in – faulty air-con, choked rest room and all. Get round this by asking your agent to incorporate a ultimate inspection clause within the possibility-to-buy letter. HDB flat patrons routinely take pleasure in this security net. "There's a ultimate inspection of the property two days before the completion of all HDB transactions. If the air-con is defective, you can request the seller to repair it," says Kelvin.
15.6.1 As the agent is an intermediary, generally, as soon as the principal and third party are introduced right into a contractual relationship, the agent drops out of the image, subject to any problems with remuneration or indemnification that he could have against the principal, and extra exceptionally, against the third occasion. Generally, agents are entitled to be indemnified for all liabilities reasonably incurred within the execution of the brokers´ authority.
To achieve the very best outcomes, you must be always updated on market situations, including past transaction information and reliable projections. You could review and examine comparable homes that are currently available in the market, especially these which have been sold or not bought up to now six months. You'll be able to see a pattern of such report by clicking here It's essential to defend yourself in opposition to unscrupulous patrons. They are often very skilled in using highly unethical and manipulative techniques to try and lure you into a lure. That you must also protect your self, your loved ones, and personal belongings as you'll be serving many strangers in your home. Sign a listing itemizing of all of the objects provided by the proprietor, together with their situation. HSR Prime Recruiter 2010. - Jon Lee; A First Course in Combinatorial Optimization; Cambridge University Press; 2004; ISBN 0-521-01012-8.
- Christos H. Papadimitriou and Kenneth Steiglitz Combinatorial Optimization : Algorithms and Complexity; Dover Pubns; (paperback, Unabridged edition, July 1998) ISBN 0-486-40258-4.
Journals
- Computational Optimization and Applications
- Journal of Computational Optimization in Economics and Finance
- Journal of Economic Dynamics and Control
- SIAM Journal on Optimization (SIOPT) and Editorial Policy
- SIAM Journal on Control and Optimization (SICON) and Editorial Policy
External links
- COIN-OR—Computational Infrastructure for Operations Research
- Decision Tree for Optimization Software Links to optimization source codes
- Global optimization
- Mathematical Programming Glossary
- Mathematical Programming Society
- NEOS Guide currently being replaced by the NEOS Wiki
- Optimization Online A repository for optimization e-prints
- Optimization Related Links
- Convex Optimization I EE364a: Course from Stanford University
- Convex Optimization – Boyd and Vandenberghe Book on Convex Optimization
- Book and Course on Optimization Methods for Engineering Design
Template:Optimization algorithms
47 year-old Environmental Consultant Lester from Port Coquitlam, really loves skydiving, property developers in singapore and cake decorating. that included taking a trip to Ancient City of Ping Yao.
Feel free to visit my blog; thewrightview.com
Template:Systems Engineering
- ↑ "The Nature of Mathematical Programming," Mathematical Programming Glossary, INFORMS Computing Society.
- ↑ W. Erwin Diewert (2008). "cost functions," The New Palgrave Dictionary of Economics, 2nd Edition Contents.
- ↑ Peter Newman (2008). "indirect utility function," The New Palgrave Dictionary of Economics, 2nd Edition. Contents.
- ↑ One of the biggest reasons investing in a Singapore new launch is an effective things is as a result of it is doable to be lent massive quantities of money at very low interest rates that you should utilize to purchase it. Then, if property values continue to go up, then you'll get a really high return on funding (ROI). Simply make sure you purchase one of the higher properties, reminiscent of the ones at Fernvale the Riverbank or any Singapore landed property Get Earnings by means of Renting
In its statement, the singapore property listing - website link, government claimed that the majority citizens buying their first residence won't be hurt by the new measures. Some concessions can even be prolonged to chose teams of consumers, similar to married couples with a minimum of one Singaporean partner who are purchasing their second property so long as they intend to promote their first residential property. Lower the LTV limit on housing loans granted by monetary establishments regulated by MAS from 70% to 60% for property purchasers who are individuals with a number of outstanding housing loans on the time of the brand new housing purchase. Singapore Property Measures - 30 August 2010 The most popular seek for the number of bedrooms in Singapore is 4, followed by 2 and three. Lush Acres EC @ Sengkang
Discover out more about real estate funding in the area, together with info on international funding incentives and property possession. Many Singaporeans have been investing in property across the causeway in recent years, attracted by comparatively low prices. However, those who need to exit their investments quickly are likely to face significant challenges when trying to sell their property – and could finally be stuck with a property they can't sell. Career improvement programmes, in-house valuation, auctions and administrative help, venture advertising and marketing, skilled talks and traisning are continuously planned for the sales associates to help them obtain better outcomes for his or her shoppers while at Knight Frank Singapore. No change Present Rules
Extending the tax exemption would help. The exemption, which may be as a lot as $2 million per family, covers individuals who negotiate a principal reduction on their existing mortgage, sell their house short (i.e., for lower than the excellent loans), or take part in a foreclosure course of. An extension of theexemption would seem like a common-sense means to assist stabilize the housing market, but the political turmoil around the fiscal-cliff negotiations means widespread sense could not win out. Home Minority Chief Nancy Pelosi (D-Calif.) believes that the mortgage relief provision will be on the table during the grand-cut price talks, in response to communications director Nadeam Elshami. Buying or promoting of blue mild bulbs is unlawful.
A vendor's stamp duty has been launched on industrial property for the primary time, at rates ranging from 5 per cent to 15 per cent. The Authorities might be trying to reassure the market that they aren't in opposition to foreigners and PRs investing in Singapore's property market. They imposed these measures because of extenuating components available in the market." The sale of new dual-key EC models will even be restricted to multi-generational households only. The models have two separate entrances, permitting grandparents, for example, to dwell separately. The vendor's stamp obligation takes effect right this moment and applies to industrial property and plots which might be offered inside three years of the date of buy. JLL named Best Performing Property Brand for second year running
The data offered is for normal info purposes only and isn't supposed to be personalised investment or monetary advice. Motley Fool Singapore contributor Stanley Lim would not personal shares in any corporations talked about. Singapore private home costs increased by 1.eight% within the fourth quarter of 2012, up from 0.6% within the earlier quarter. Resale prices of government-built HDB residences which are usually bought by Singaporeans, elevated by 2.5%, quarter on quarter, the quickest acquire in five quarters. And industrial property, prices are actually double the levels of three years ago. No withholding tax in the event you sell your property. All your local information regarding vital HDB policies, condominium launches, land growth, commercial property and more
There are various methods to go about discovering the precise property. Some local newspapers (together with the Straits Instances ) have categorised property sections and many local property brokers have websites. Now there are some specifics to consider when buying a 'new launch' rental. Intended use of the unit Every sale begins with 10 p.c low cost for finish of season sale; changes to 20 % discount storewide; follows by additional reduction of fiftyand ends with last discount of 70 % or extra. Typically there is even a warehouse sale or transferring out sale with huge mark-down of costs for stock clearance. Deborah Regulation from Expat Realtor shares her property market update, plus prime rental residences and houses at the moment available to lease Esparina EC @ Sengkang - ↑
20 year-old Real Estate Agent Rusty from Saint-Paul, has hobbies and interests which includes monopoly, property developers in singapore and poker. Will soon undertake a contiki trip that may include going to the Lower Valley of the Omo.
My blog: http://www.primaboinca.com/view_profile.php?userid=5889534 - ↑ Lionel Robbins (1935, 2nd ed.) An Essay on the Nature and Significance of Economic Science, Macmillan, p. 16.
- ↑ A. K. Dixit ([1976] 1990). Optimization in Economic Theory, 2nd ed., Oxford. Description and contents preview.
- ↑ A.G. Malliaris (2008). "stochastic optimal control," The New Palgrave Dictionary of Economics, 2nd Edition. Abstract.
- ↑ Julio Rotemberg and Michael Woodford (1997), "An Optimization-based Econometric Framework for the Evaluation of Monetary Policy.NBER Macroeconomics Annual, 12, pp. 297-346.
- ↑ From The New Palgrave Dictionary of Economics (2008), 2nd Edition with Abstract links:
• "numerical optimization methods in economics" by Karl Schmedders
• "convex programming" by Lawrence E. Blume
• "Arrow–Debreu model of general equilibrium" by John Geanakoplos. - ↑ Template:Cite web
- ↑ Gharib Shirangi, M., History matching production data and uncertainty assessment with an efficient TSVD parameterization algorithm, Journal of Petroleum Science and Engineering, http://www.sciencedirect.com/science/article/pii/S0920410513003227